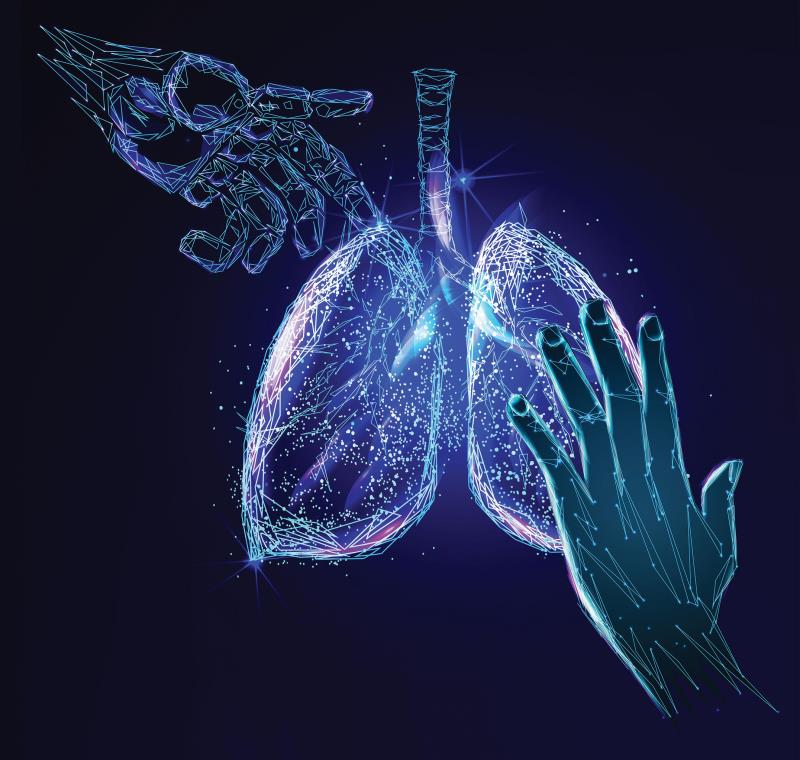
An artificial intelligence (AI)–powered spatial analytical tool for predicting the effectiveness of immune checkpoint inhibitors (ICIs) in advanced non-small-cell lung cancer (NSCLC) has been developed by researchers from the Chinese University of Hong Kong (CUHK), Seoul National University, Sungkyunkwan University, Ajou University, and AI start-up Lunit.
“ICIs are standard of care for advanced NSCLC with positive programmed death ligand-1 [PD-L1] expression,” said Professor Tony Shu-Kam Mok of the Department of Clinical Oncology, CUHK. “Treatment outcome with PD-L1 checkpoint inhibitors may vary depending on the patient’s tumour microenvironment, but there is currently no standard biomarker addressing this.”
“Tumour infiltrating lymphocytes [TILs], in theory, are the main activator of antitumour immunity, which could be a promising biomarker for predicting treatment outcomes with ICIs,” added Mok. “However, the current quantification method is labour-intensive and relies on spatial distribution in whole-slide images [WSIs], limiting utility and objectivity.”
The AI-powered spatial TIL analyzer is capable of segmentation and quantification of multiple histologic components from WSIs, such as TILs, cancer epithelium (CE), and cancer stroma (CS). Three immune phenotypes (IPs), namely immune-excluded (TIL density in CE; below threshold of 106/mm2; TIL density in CS, above threshold of 357mm2), inflamed (TIL density in CE, above threshold), and immune-desert (TIL density in CS, below threshold) phenotypes, are defined through deep learning–based AI model training. [J Clin Oncol 2022;doi:10.1200/JCO.21.02010]
At the WSI level, there was a significant positive correlation between tumour proportion score (TPS; current method of PD-L1 measurement) as determined by the AI model and control TPS analyzed by pathologists (p<0.001). “We adopted a supervised learning model using original images with independent annotated markings of CE, CS, and TILs as assessed by a panel of experienced pathologists …This meticulous supervision enabled pixel-based spatial analysis of TILs in reference to CE and CS, which is essential for objective quantification of IP and eventual classification of IP,” explained the researchers.
To validate IPs as a complementary biomarker, the researchers analyzed patients with advanced NSCLC treated with ICI monotherapy in a retrospective proof-of-concept cohort study (n=518; mean age, 65.0 years; male, 75.9 percent).
Results of the study showed that the tumour response rate to ICIs in patients with inflamed IP was higher than that in patients with immune-excluded and immune-desert IPs (26.8 percent vs 11.5 percent vs 11.2 percent). Similarly, their median progression-free survival (PFS) with ICIs was significantly longer than that in patients with immune-excluded and immune-desert IPs (4.1 months vs 2.2 months vs 2.4 months; both p<0.001), as was overall survival (24.8 months vs 14.0 months vs 10.6 months; both p<0.05), indicating that IP may be a promising biomarker for predicting treatment outcomes.
According to the researchers, 42.5 percent of patients with PD-L1 TPS 1–49 percent were classified as having inflamed IP, which appeared to be higher than the corresponding reported rate of 14.8 percent from the KEYNOTE 001 study, suggesting that more potential ICI responders could be found with the help of the AI-powered spatial TIL analyzer. [N Engl J Med 2015;372:2018-2028]
“To our knowledge, this is the first study on AI-powered automated TIL analysis in advanced NSCLC,” concluded the researchers. “In this study, we have proved that the AI-powered spatial TIL analyzer is capable of predicting clinical outcome with ICIs in patients with advanced NSCLC. This may serve as a complementary biomarker to TPS, especially for the subgroup of patients with PD-L1 TPS 1–49 percent.”