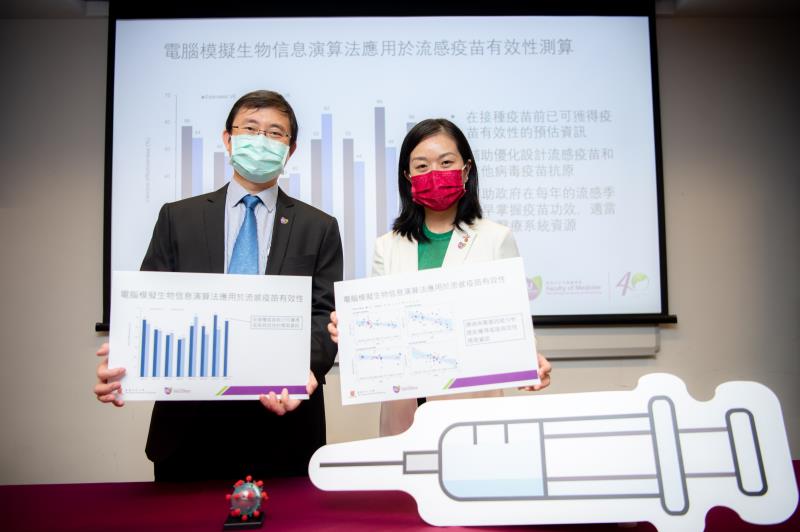
Experts at the Chinese University of Hong Kong (CUHK) have developed a bioinformatic model for accurate, real-time in silico prediction of influenza vaccine effectiveness (VE) for influenza A/H1N1pdm09, A/H3N2 and B prior to mass vaccination, potentially informing the influenza vaccine selection strategy.
Evaluation of effectiveness of seasonal influenza vaccine is commonly conducted in longitudinal or retrospective studies, causing difficulty in enabling timely assessment of VE prior to mass vaccination during an influenza epidemic. [Vaccine 2021;39:1030-1034]
“Quantifying the genetic distance between circulating viral strains and vaccine strains is the key to assessing influenza VE. Our team previously developed a computational approach to identify the key amino acid substitutions attributable to mutation of influenza virus. Genetic mismatch between circulating strains and vaccine strains on these key viral mutation sites have been captured,” explained Professor Benny Chung-Ying Zee of Centre for Clinical Research and Biostatistics, CUHK.
“Our current study demonstrated significant associations between genetic mismatch and VE [for influenza A/H1N1pdm09, A/H3N2 and B], enabling real-time in silico prediction of influenza VE,” said Zee. “This is of high significance to public health as it could facilitate optimization of vaccine formulation and assessment of vaccine products for the local population in a real-time manner during epidemics, given that regions with year-round influenza activity, such as tropical or subtropical regions, may have uncertainty in selecting vaccine products containing strains from the Northern or Southern Hemisphere.”
Results of the study showed significant reduction of VE with genetic mismatch, measured by effective mutation distance (EMD), on the hemagglutinin (HA) gene of influenza A/H1N1pdm09 (6.59 percent; p=0.017; R2=0.417) and influenza A/H3N2 (2.28 percent; p=0.0039; R2=0.514).
Modelling framework yielded greater performance for datasets from the UK and Germany than those from Hong Kong, likely due to the high viral diversity in China with greater variation in pairwise genetic distance from vaccine strains.
Similarly, EMD on the neuraminidase (NA) gene of influenza A H1N1pdm09 was significantly associated with VE (p=0.006; R2=0.512). The absence of significant association between EMD on NA of influenza A/H3N2 and VE (p=0.67, R2=0.0159) was likely due to subtype-specific effect or absence of NA specification in current vaccine design.
Greater association with VE was shown with EMD on combined HA and NA of influenza A/H1N1 (p=0.0087; R2=0.48) and A/H3N2 (p=0.0034; R2=0.525), with a p value of 0.0072 for the association between EMD of all influenza types (influenza A/H1N1pdm09, A/H3N2 and B) and VE (R2=0.616).
“Our findings showed that the computational model allowed real-time monitoring of genetic distance between circulating influenza viruses and vaccines on a large scale, enabling timely updates on the expected effectiveness of vaccine products in a local population, potentially informing the influenza vaccine selection strategy,” said Professor Maggie Haitian Wang of the Jockey Club School of Public Health and Primary Care, CUHK.
The researchers retrieved data on genetic sequences of influenza A/H1N1pdm09, A/H3N2 and B from the National Centre for Biotechnology Information (NCBI). The bioinformatic model was developed and trained using 3,300 HA and NA protein sequences from the US during the 2009/10 and 2018/19 influenza seasons and validated with those sequences isolated in Canada, UK, Germany, Spain and Hong Kong during the 2010/11 and 2018/19 influenza seasons.