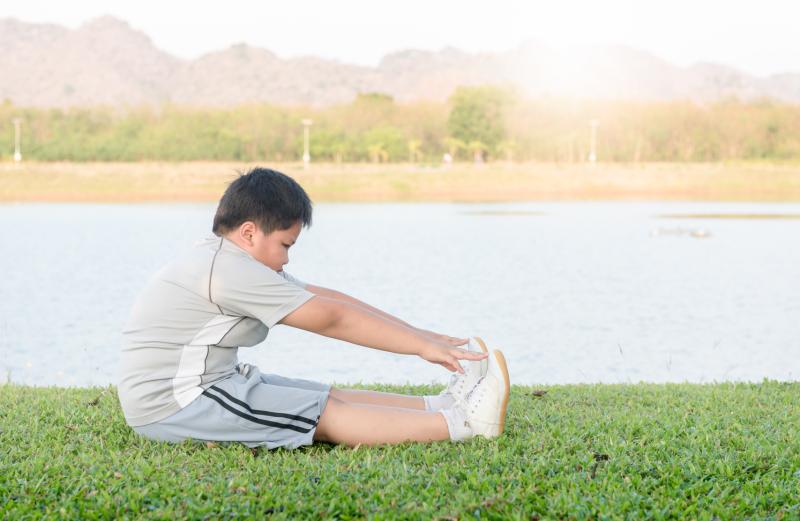
Machine learning (ML) outperforms existing paediatric clinical screening guidelines in identifying prediabetes and diabetes mellitus (preDM/DM) among young people, a recent study has found.
Drawing from the National Health and Nutrition Examination of the USA, the researchers predicted preDM/DM in 2,858 young people using a widely used clinical screening guideline—published by the American Diabetes Association and endorsed by the American Academy of Paediatrics (ADA/AAP)—as well as with ML classifiers. Diagnostic biomarkers designated by the ADA were used as the gold standard.
According to the ADA biomarker criterion, approximately 29 percent of the youth study population had preDM/DM, whereas the ADA/APA clinical guideline found the prevalence to be 35.5 percent.
The ADA/APA guideline could correctly identify 43.1 percent of the youth with preDM/DM, with a positive predictive value (PPV) of 35.2 percent. Agreement was poor between the clinical guideline and the ADA biomarker criteria (kappa coefficient, 0.1, 95 percent confidence interval [CI], 0.06–0.14; p<0.0001).
ML classifiers for preDM/DM were generated using ten established algorithms and a five-fold cross-validation setup, and in accordance with same guidelines as outlined in the ADA/APA criteria. The ML methods performed comparably, or in some instances, better than the clinical guideline at identifying youth with preDM/DM.
“Our demonstration that the guideline did not perform well … points to the need for additional work to develop a simple yet accurate screener for youth diabetes risk,” the researchers said. “In particular, our investigation of ML methods applied to these data demonstrates the promise of automated data-driven methods for developing such screeners.”
“Future work includes the use of more advanced ML methods applied to a wider range of clinical and behavioural health data available in NHANES to build better predictive tools for assessing preDM/DM risk,” they added.