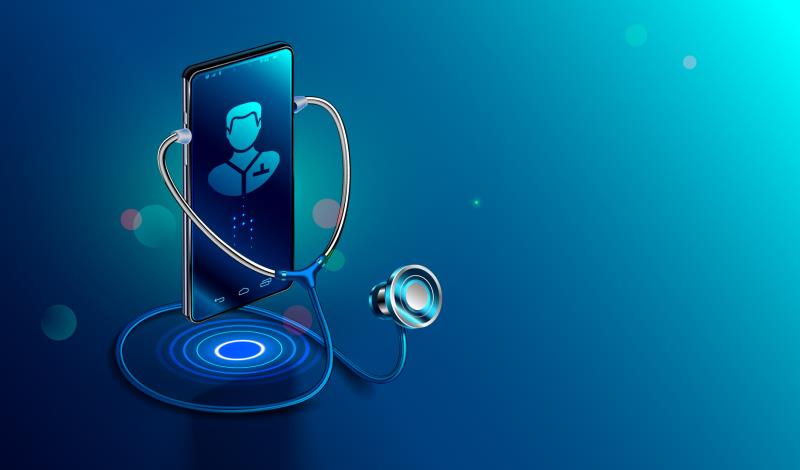
A novel smartphone app that uses machine learning algorithms to analyse facial expressions, speech patterns, and arm movements can detect acute stroke and Bell’s palsy with an accuracy matching that of neurologists, as shown in a study.
Named FAST-AI (standing for face, arm, speech, and time to call for help), the app integrates multiple AI models. First is a facial imaging model that analyses smartphone camera-captured images against 90 landmark facial points for subtle asymmetries that might indicate a stroke as opposed to Bell’s palsy. Another is an arm movement model, called “grasp agnostic,” which extracts data from the smartphone’s built-in sensors to detect signs of weakness while holding and moving the phone. Third is a speech pattern model that processes recorded voice based on frequency analysis and Mel Frequency Cepstral Coefficients (MFCC) to identify abnormal or slurred speech.
Lead study investigator Dr Radoslav Raychev of the University of California Los Angeles in California, US, argued that campaigns to improve stroke recognition using the “FAST” method have seen minimal success due to human memory limitations. The method, he said, relies on people to remember and apply the acronym.
“We decided to automate FAST using smartphones because they are extremely common in peoples’ day-to-day routines across the world,” Raychev stated.
Near-expert precision
The AI models in the app were trained and tested using data from 400 stroke patients (median age, 69 years; women, 45.3 percent) and 71 healthy controls. Of the patients, 66 percent had ischaemic stroke, 5.5 percent had haemorrhagic stroke, and 11 percent had Bell’s palsy. Data from 280 patients were used in the training phase, and data from 120 were used in the testing and validation phase.
The performance of the AI models for detecting stroke versus no stroke and stroke versus Bell’s palsy was evaluated against the clinical impressions of neurologists. All of the tests for stroke patients were conducted within 72 hours of symptom onset.
FAST-AI was able to detect stroke with 99 percent sensitivity and 90 percent specificity, as well as Bell’s palsy with 91 percent sensitivity and 81 percent specificity.
Looking at the individual AI models, the facial model achieved a sensitivity of 92 percent and specificity of 78 percent at identifying which side of the face had been affected by stroke. It was also able to differentiate between asymmetry due to stroke and asymmetry due to Bell’s palsy.
Meanwhile, the arm movement model had 78 percent sensitivity and 79 percent specificity for detecting arm weakness based on motion direction, height, and acceleration changes, independent of grip and smartphone orientation.
Finally, the speech pattern model enabled the detection of slurred speech based on Mel-frequency cepstrum coefficients with 75 percent sensitivity and 75 percent specificity. This model was initially trained and tested on Bulgarian speech data and is set to be adapted to languages such as English and Spanish, among others, according to Raychev.
“Our first iteration will be a clinician aid and rolled out to EMTs, paramedics, and other responders to expand their neurological examination capabilities,” Raychev said.
“Our ultimate goal is to develop platform-independent, consumer-facing technology that can be used any time there is any question or concern of a stroke by active self-testing, or even as an ambient monitoring tool in smart home systems, autonomous driving vehicles, video conferencing and more,” he added.