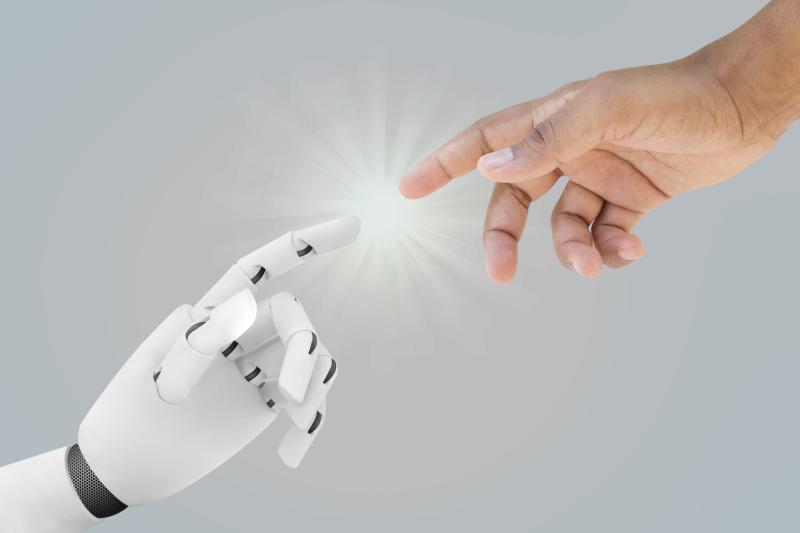
A machine learning model that incorporates artificial intelligence (AI) techniques to predict the blood concentration of quetiapine is feasible for clinical medication guidance in patients with schizophrenia and depression, reports a real-world study.
A team of investigators obtained a total of 650 cases of quetiapine therapeutic drug monitoring (TDM) data from 483 patients at the First Hospital of Hebei Medical University in China from 1 November 2019 to 31 August 2022. They carried out univariate analysis and sequential forward selection (SFS) to screen the important variables that affected quetiapine TDM.
Following a tenfold cross validation, the algorithm with the optimal model performance was chosen among nine models for the prediction of quetiapine TDM. The Shapley Additive Explanation was used to interpret the model.
In univariate analysis and SFS, four variables (ie, daily dose of quetiapine, type of mental illness, sex, and CYP2D6 competitive substrates) were selected (p<0.05) to establish the models. The investigators picked the CatBoost algorithm with the best predictive ability (mean R2, 0.63; RMSE, 137.39; MAE, 103.24) to predict quetiapine TDM among the nine models.
The mean accuracy of the predicted TDM within ±30 percent of the actual TDM was 49.46 percent, while that of the recommended therapeutic range (200‒750 ng/mL-1) was 73.54 percent.
Notably, the CatBoost model demonstrated slightly higher accuracy within ±100 percent of the actual value than the PBPK model in an earlier study.