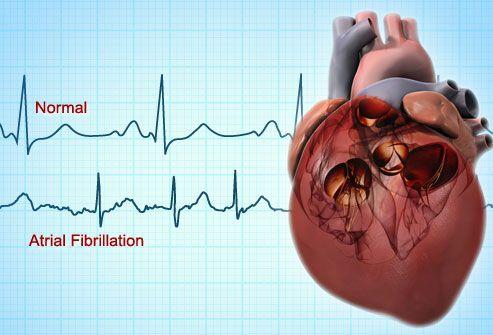
Using a resting 12-lead digital electrocardiogram (ECG), deep neural networks (DNNs) can be trained to predict the risk of new-onset atrial fibrillation (AF) within a year in people with no prior history, according to a recent study.
“This predictive performance surpasses that of currently available clinical models, persists even within ECGs interpreted as normal, and is associated with significant hazard for AF development over the next 30 years,” the researchers said.
DNNs were trained to predict 1-year new-onset AF using over 1.6 million resting 12-lead digital ECG traces. ECGs were obtained from more than 430,000 patients who had no history of AF, and were collected from 1984 to 2019.
When trained on ECG traces alone, the model yielded high predictive value for new-onset AF within 1 year, with an area under the receiver operating curve (AUROC) of 0.83. At the same time, the area under the precision recall curve (AUPRC), which describes the tradeoff between true positive rate and the positive predictive value, was 0.21. [Circulation 2021;doi:10.1161/CIRCULATIONAHA.120.047829]
Incorporating age and sex into the model slightly boosted the DNN’s performance, improving AUROC and AUPRC to 0.85 and 0.22, respectively.
CHARGE-AF* risk scores were available for 65 percent of the patients. In this subset, the DNN model with ECG and age and sex performed better than CHARGE AF both in terms of AUROC (0.84 vs 0.79) and AUPRC (0.20 vs 0.12). Notably, DNNs continued to show high predictive value in the subgroup of ECGs that were clinically normal.
Moreover, the 1-year AF risk as estimated by the DNN models correlated with the likelihood of developing AF over a 30-year follow-up (DNN with ECG alone: hazard ratio [HR], 6.7, 95 percent confidence interval [CI], 6.4–7.0; DNN with ECG, age, and sex: HR, 7.2, 95 percent CI, 6.9–7.6). Adjusting for age and sex weakened the magnitude of the interaction but did not attenuate significance.
To simulate real-world settings, the researchers trained a new model on ECG traces collected before 2010 and tested its predictive ability in traces obtained between 2010 and 2014. They found that DNNs performed well at predicting new-onset AF within a year (AUROC, 0.83; AUPRC, 0.17), with sensitivity and specificity values of 69 percent and 81 percent, respectively. The number needed to screen in order to find one AF case at 1 year was 9.
“Preliminary data simulating a real-world deployment scenario demonstrate that using this tool identifies a high-risk population for new-onset AF that can be targeted for increased screening and may prove useful for helping to prevent AF-related strokes,” the researchers said.
“A prospective clinical trial is needed to confirm how many strokes can be prevented using a screening strategy on the basis of enhanced monitoring as a result of an AF risk prediction,” they added.
*Cohorts for Aging and Research in Genomic Epidemiology