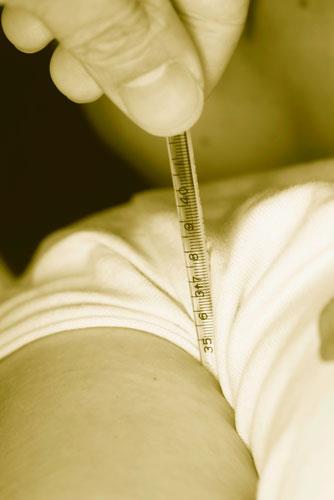
The gradient boosting decision tree (GBDT) model appears more reliable than previous models in predicting intravenous immunoglobulin (IVIG)-resistant Kawasaki disease (KD), reports a study.
A team of investigators developed a predictive model for IVIG-resistant KD based on GBDT to identify children with such resistance and improve treatment to prevent adverse events. They collected the case data of KD patients who were hospitalized in the Pediatric Department of Lanzhou University Second Hospital from October 2015 to July 2020.
Children with KD were divided into IVIG-resistant and IVIG-responsive groups. GBDT was applied to determine the influencing factors of IVIG-resistant KD and to create a prediction model. The investigators then compared this with previous models.
To construct the GBDT model, 80 percent of the obtained data were used as the test set, while the remaining 20 percent were used as the validation set. The verification set was then used to fine-tune the hyperparameters in GBDT learning. The model displayed its best performance with a hyperparameter tree depth of 5.
The area under the curve of the GBDT model constructed using the best parameters was 0.87 (95 percent confidence interval [CI], 0.85‒0.90), with a sensitivity of 72.62 percent, a specificity of 89.04 percent, and an accuracy of 61.65 percent.
“The contribution degree of each feature value to the model was total bilirubin, albumin, C-reactive protein, fever time, and Na in order,” the investigators said.