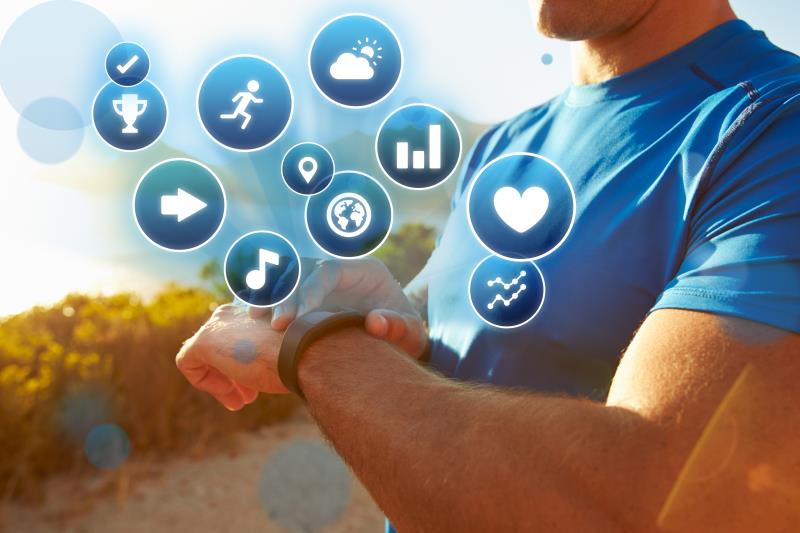
Using free-living physical activity data from wrist-worn accelerometers, machine learning algorithms can be trained to differentiate people type 2 diabetes (T2D) from those with normal glucose levels, according to a recent study.
“Using data from the UK Biobank, this study supports the hypothesis that individuals with diagnosed T2D exhibit physical activity patterns that are significantly different from those of normoglycaemic controls, thus providing novel ways to detect T2D, that is, through appropriate analysis of physical activity patterns,” the researchers said.
Through a combined analysis of self-reported data and the electronic health records, a total of 3,103 eligible T2D patients were identified from the UK Biobank study. Physical activity data were collected using triaxial wrist-worn devices worn for 1 week.
Machine learning algorithms were then trained on two data sets which compared physical activity levels between T2D vs normoglycaemic controls expected to have no (n=11,019) or high (n=3,355) impairments in their ability to perform usual physical activities.
When data were aggregated to the level of a 24-hour day, there appeared to be no difference in physical activity between T2D patients and both normoglycaemic control groups. In approximates, they all spent 5 percent of the time on moderate activity, 42 percent on sedentary activity, 38 percent asleep, 5 percent on light tasks, and 10 percent walking. [JMIR Diabetes 2021;6:e23364]
However, looking at activity bouts rather than just the percentage of time dedicated to each activity revealed differences that could potentially facilitate discrimination between groups.
“An activity bout is defined as a single epoch or an uninterrupted consecutive series of epochs in which a single activity type is performed. The length of a bout refers to the many 30-second epochs for which each bout is performed,” the researchers explained.
In particular, normoglycaemic controls with no impairments tended to have better sleep, with longer and more frequent sleep bouts than T2D patients.
Subsequent binary classification analysis further showed that device-measured physical activity features combined with sociodemographic and lifestyle factors could reliably differentiate T2D patients from normoglycaemic controls.
However, such data may be inherently noisy due to its free-living nature. Models constructed in training sets including controls that were not physically impaired performed better; training sets that enrolled controls that had high impairments for normal activity had weaker discriminative ability.
“[W]e have demonstrated the benefits of extracting a more objective and granular representation of physical activity from raw accelerometry traces data, namely, by activity type and time of day or sleep time,” the researchers said.
“Using these features, either on their own or in combination with a selected set of sociodemographic, anthropometric, and lifestyle variables, we have shown that appropriately trained machine learning models were able to discriminate between the two cohorts with good predictive accuracy,” they added.
More practically, the present findings also suggest “that it may be possible to use continuous or periodic self-monitoring of individuals at risk of T2D, specifically those in a prediabetes state, for screening and early detection of disease progression,” they said.