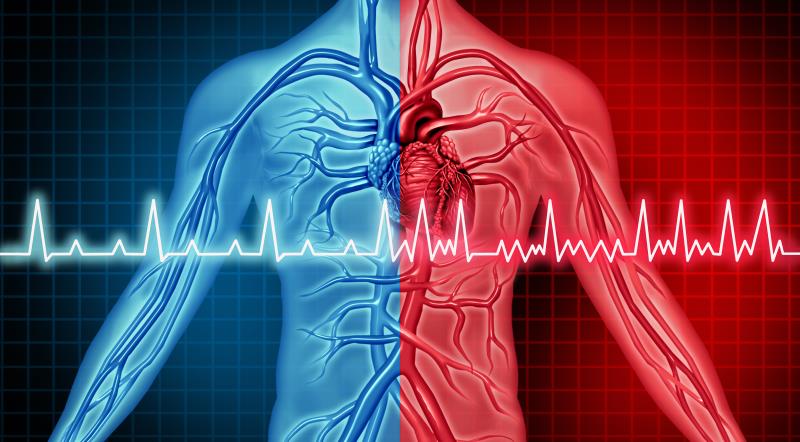
Use of machine learning (ML) can help pinpoint novel factors that contribute to an increased risk of atrial fibrillation (AF) in non-White patients with heart failure (HF), suggests a study. Specifically, social factors such as living alone may significantly heighten the AF risk.
“The integration of ML into clinical practice is mutually beneficial. It offers clinicians improved clinical workflow and diagnostic accuracy and cost-effectiveness. Clinicians offer ML the essential exposure it needs to learn complex clinical case management. This, in turn, increases critical mass and encourages buy-in from multiple disciplinary approaches,” the researchers said.
Patient-level data from the Warfarin and Aspirin in Patients with Heart Failure and Sinus Rhythm (WARCEF) trial were used in this study. The research team applied four ML models combined with logistic regression and cluster analysis post hoc to determine the predictors of AF in patients with HF.
In logistic regression, AF risk was 1.75-fold higher among White divorced patients compared with White patients with other marital statuses. Similar analysis revealed that non-White patients who live alone had a 2.58-fold greater risk than those not living alone. [Am J Med 2023;136:1099-1108.E2]
Social isolation
Analysis using ML also identified marital status and living alone as significant risk factors of AF. Additionally, ML algorithms and cluster analysis identified two distinct clusters: White and non-White ethnicities. Such findings highlighted the impact of social factors on health.
“Our analysis has shown that social factors, such as living alone, may disproportionately increase the risk of AF in the under-represented non-White patient group with HF, highlighting the need for more studies focusing on stratification of multiracial cohorts to better uncover the heterogeneity of AF,” the researchers said.
Previous studies have analysed the effect of race and ethnicity as part of the social determinant in AF incidence. An interesting finding showed that although risk factors for AF are highly prevalent, non-White patients have a lower AF prevalence. Its aetiology is also unclear because of its complex nature. [Nat Rev Cardiol 2021;18:763-773; Future Cardiol 2009;5:547-556]
“Apart from recognizable factors such as household income, which was not recorded within the dataset, we have shown that living alone had a greater impact on ethnic minorities in terms of developing AF,” the researchers said. [Circ Arrhythm Electrophysiol 2018;11e006350]
Earlier studies have also reported the impact of social isolation on cardiovascular mortality. The current study confirmed this, showing that living alone disproportionately affected non-White patients of the WARCEF trial. [Am J Public Health 2013;103:2056-2062; Stroke 2008;39:768-775]
AF detection
ML technologies, when implemented effectively, can be a useful tool in the early and accurate detection of AF, which then allows precise stratification of patient groups.
“The health impact of experiences of loneliness among people from Black, Asian, and minority ethnic groups seems to have far-reaching implications, including an apparent higher risk of developing AD,” the researchers said.
“This needs to be further investigated and understood so that a series of recommendations for national policymakers, local authorities, and health service providers can be put forward, and people experiencing loneliness can get the help and support they need,” they added.
The current study was limited by its post hoc design and small sample size. In addition, no systematic screening for AF was performed in the WARCEF trial, potentially leading to an underestimation of patients who developed AF.