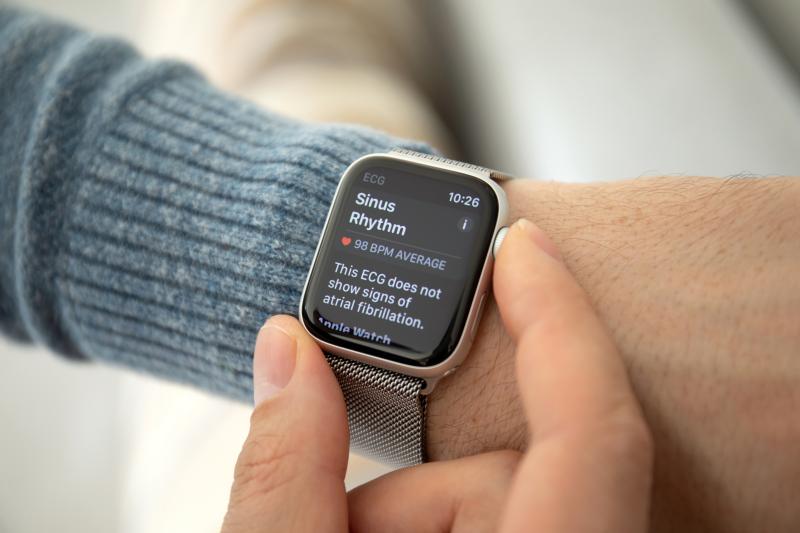
Digital biomarkers collected by smartphones and wearable sensors may help detect behavioural and physiological changes signalling symptom burden in pancreatic cancer patients before and after surgery, according to a recent study.
“The purpose of this prospective longitudinal study was to evaluate passive smartphone and wearable sensor features as predictors of symptom burden in perioperative patients undergoing pancreatic surgery,” the researchers said. “Results suggest that machine learning models developed using mobile sensor data were more accurate than nonsensor-based baseline models in predicting whether the next-day patient-reported overall symptom burden would be higher than average for that patient.”
Forty-four patients with enough device-collected and self-reported symptom data were enrolled into the study. Smartphones and fitness trackers collected day-level behavioural features related to mobility, activity, sleep, screen time, heart rate, and communication; at the same time, symptom ratings were self-reported. Data collection started at least 2 weeks before surgery, continuing through inpatient recovery and lasting for up to 60 days after discharge.
Using the tree-based algorithm light gradient boosting machine (lightGBM), the researchers saw that models using smartphone and wearable sensor data could correctly predict whether patients would have high symptom burden in the following day with an accuracy of 73.5 percent. The area under the curve (AUC) was 0.772. [JMIR Cancer 2021;7:e27975]
Notably, the lightGBM model, taking into account all device-collected features, could also predict next-day diarrhoea, fatigue, and pain well, with accuracy ratings of 79.0 percent (AUC, 0.834), 75.8 percent (AUC, 0.803), and 79.6 percent (AUC, 0.835), respectively.
To put the performance of their constructed lightGBM model into better context, the researchers compared it against three baselines models: majority class, random weighted classifier, and decision tree using nonsensor features.
They found that for the prediction of overall next-day symptom burden, lightGBM was more accurate that the baseline models, which yielded accuracy ratings ranging from 54.1 percent to 67.5 percent. The same was true for diarrhoea (accuracy from 56.0 percent to 73.2 percent), fatigue (from 54.3 percent to 67.0 percent), and pain (from 58.4 percent to 74.4 percent).
Looking at each device-collected feature individually, the researchers found that the most recent symptom score, the number of days since surgery, and the average symptom burden score to date were the most impactful factors for predicting overall symptom burden. In comparison, location features were crucial for predicting diarrhoea, step features for fatigue, and sleep features for pain.
“Because wearable and smartphone sensor data can be collected continuously as patients go about their daily lives, requiring minimal effort or attention from patients or their caregivers, mobile sensing offers an opportunity for long-term remote patient monitoring over months or years of cancer treatment and survivorship,” the researchers said.
Findings of the present study support such an approach, they added, noting the particular importance of features related to physical activity, heart rate, and location. Nevertheless, more studies are needed to validate the value of mobile sensing in cancer patients.
“With a larger outpatient sample using their own smartphones, we hope to improve upon the models developed here and to use real-time next-day symptom predictions to deliver more timely and personalized symptom management support,” the researchers said.