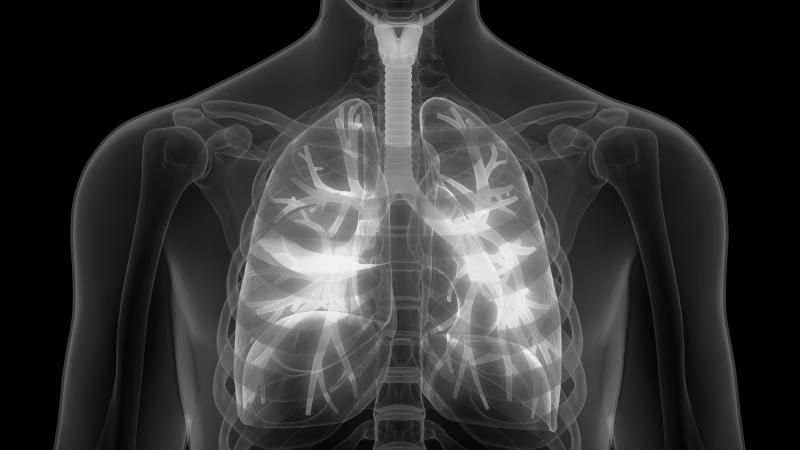
Prediction models for the diagnosis and prognosis of the novel coronavirus disease (COVID-19) have sprung up and appear to show excellent discriminative performance. However, a recent study has found that these models are at high risk of bias due to nonrepresentative selection of control patients, exclusion of those who had not experienced the event of interest by end of study, and model overfitting.
“Therefore, their performance estimates are likely to be optimistic and misleading,” the researchers said. “Immediate sharing of well-documented individual participant data from COVID-19 studies is needed for collaborative efforts to develop more rigorous prediction models and validate existing ones.”
In this rapid systematic review and critical appraisal, studies that developed or validated a multivariable COVID-19–related prediction model were searched from PubMed and Embase through Ovid, Arxiv, medRxiv and boRxiv up to 24 March 2020.
Data were independently extracted by at least two researchers using the critical appraisal and data extraction for systematic reviews of prediction modelling studies (CHARMS). Risk of bias was evaluated using the prediction model risk of bias assessment tool (PROBAST)
Of the 2,696 titles screened, 27 studies including 31 prediction models met the eligibility criteria. Three of these models were developed for predicting hospital admission from pneumonia and other events (as proxy outcomes for COVID-19 pneumonia) in the general population. [BMJ 2020;369:m1328]
Eighteen diagnostic models were used to detect COVID-19 infection, of which 13 were machine learning based on computed tomography scans. Ten prognostic models predicted mortality risk, progression to severe disease or length of hospital stay. Of the 27 studies, only one used data from outside of China.
Age, body temperature, and sign and symptoms were some of the most commonly reported predictors for the presence of COVID-19 in patients with suspected disease. For severe prognosis in confirmed cases, the most common predictors reported included age, sex, C-reactive protein, lymphocyte count, lactic dehydrogenase and features derived from computed tomography scans.
In prediction models for the general population, C-index estimates had a range of 0.73–0.81 (reported for all three models). In diagnostic and prognostic models, such estimates ranged from 0.81 to >0.99 (reported for 13 of 18 models) and from 0.85 to 0.98 (reported for six of 10 models), respectively.
“All studies were rated at high risk of bias, mostly because of non-representative selection of control patients, exclusion of patients who had not experienced the event of interest by the end of the study, and high risk of model overfitting,” the researchers said.
Quality of reporting also differed significantly between studies, and most reports failed to include a description of the study population of intended use of the models. In addition, calibration of predictions was not always evaluated.
“When building a new prediction model, we recommend building on previous literature and expert opinion to select predictors, rather than selecting predictors in a purely data driven way,” the researchers noted. “[T]his is especially true for datasets with limited sample size.” [Stat Methods Med Res 2019;28:2455-2474; https://link.springer.com/chapter/10.1007/978-3-030-16399-0_16]