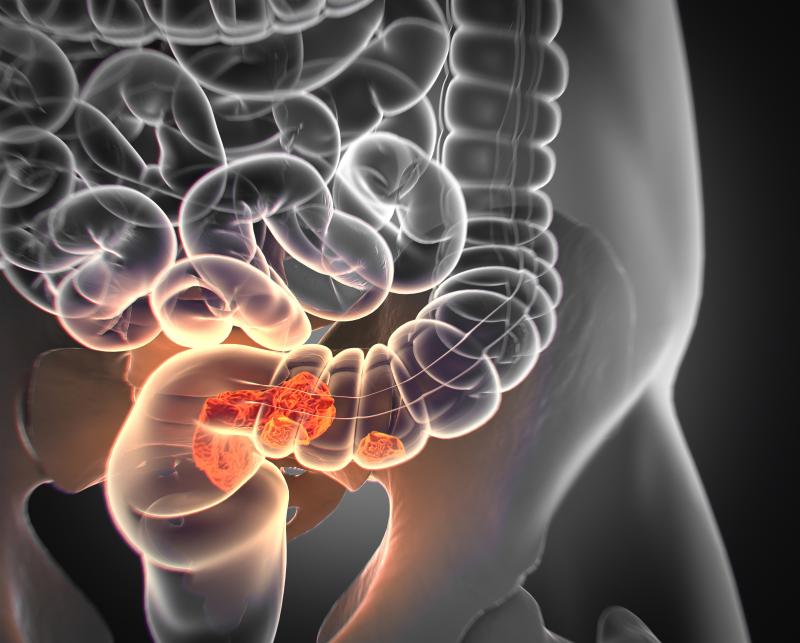
An artificial neural network (ANN) model performs better than current clinical guidelines at identifying lymph node metastasis in patients with T1 colorectal cancer (CRC), according to a new Japan study. Using artificial intelligence (AI) may be a more efficient approach of picking out patients who need additional surgery after endoscopic resection.
“To our knowledge, this is the first multicentre study to investigate the use of AI in stratifying the risk of lymph node metastasis (LNM), using such a large data set,” the researchers said. “We have shown that AI can potentially predict LNM in T1 CRCs and may be useful in decision-making as to whether additional surgical resection is indicated after endoscopic resection of T1 CRCs.”
The ANN algorithm was trained on a cohort of 3,134 T1 CRC patients, using data points such as age, sex, tumour size, location, and morphology, histologic grade, and lymphatic or vascular invasion status.
The trained model yielded an area under the curve (AUC) for LNM of 0.78. In comparison, AUC for US guidelines was 0.67; the resulting difference was statistically significant (p<0.001). [Gastroenterology 2021;160:1075-1084.e2]
When setting a fixed sensitivity threshold of 79 percent, the ANN model demonstrated a 10-percent higher specificity than the US guidelines (64.7 percent vs 54.7 percent).
The researchers then validated the algorithm on an external and independent cohort of 939 T1 CRC patients. As in the training cohort, the ANN model was superior to the US guidelines in terms of identifying LNM, with corresponding AUCs of 0.83 and 0.73; p<0.001).
When focusing on the subgroup who had undergone initial endoscopic resection, the ANN algorithm continued to be significantly better than US guidelines at predicting LNM (AUC, 0.84 vs 0.77; p=0.005).
“To reduce unnecessary surgical resections, we used AI to build a model to identify T1 colorectal tumours at risk for metastasis to lymph node and validated the model in a separate set of patients,” the researchers said.
The researchers also tested the algorithm against the Japanese guidelines for LNM. In the validation cohort, ANN was also proved to be significantly better for LNM detection in T1 CRC (AUC, 0.83 vas 0.57; p<0.001).
Limiting analysis to the subgroup of patients who had undergone initial endoscopic resection likewise did not meaningfully alter the results, with ANN displaying significantly higher predictive capacity than the Japanese guidelines (AUC, 0.84 vs 0.61; p<0.001).
“The ANN digital model imitates the functional structure of a biological neural network, utilizes large-scale nonlinear parallel processing, and has strong adaptability,” the researchers explained. “Because the ANN model does not restrict the distribution of data, it can make full use of the data information. The ANN model has strong fault tolerance, so it can be used widely for prediction and analysis of many kinds of data.”
“Together, these findings suggested that ANN, which allows nonlinear separation, will be suitable as a prediction model because the occurrence of LNM in T1 CRC is a complicated event,” they said, adding that future prospective studies are needed to validate the use of the model in clinical practice.