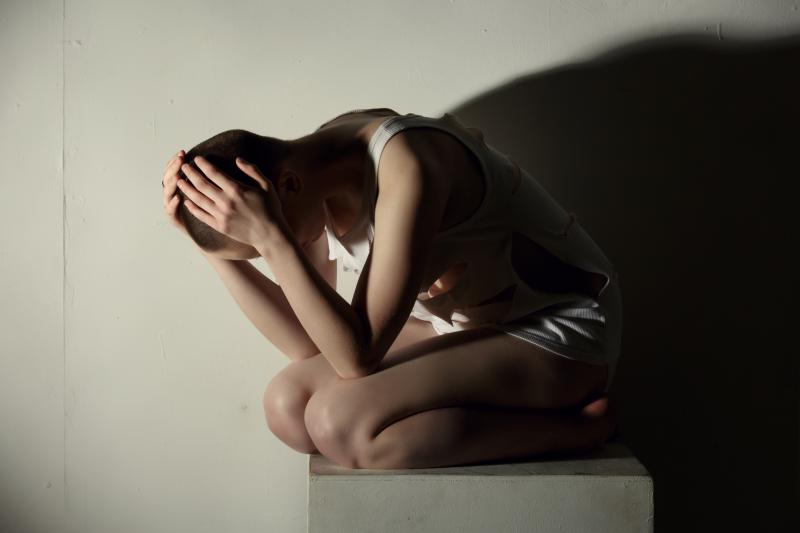
Wearable biosensors and machine learning algorithms show promise in predicting impending aggressive behaviour in youths with autism who are undergoing psychiatric treatment, offering opportunities for intervention and prevention.
In a study, a machine learning model, which was trained using time-stamped mobile data and physiological signals from wearable devices, was able to predict self-injurious behaviour, emotion dysregulation (ie, tantrums and meltdowns), and aggression toward others.
Logistic regression consistently emerged as the best-performing classifier, according to the investigators. For example, logistic regression predicted aggressive behaviour 3 minutes before onset with a mean area under the receiver operating characteristic curve (AUROC) of 0.80 (95 percent confidence interval [CI], 0.79–0.81). [JAMA Netw Open 2023;6:e2348898]
“Additionally, our results illustrated the ability to discriminate between different types and intensities of aggressive behaviour. However, collapsing these behaviours into [a single] class was associated with better detection performance and lower false-positive rates,” the investigators said.
Of the three aggressive behaviours evaluated, self-injurious behaviour was the most predictable. However, the investigators acknowledged that this may have resulted from a power issue considering that that self-injurious behaviour episodes were 1.93 and 6.43 times more frequent in the study sample than episodes of emotion dysregulation and aggression toward others, respectively.
The generalizability and reliability of the model were verified using approaches that left out specific individuals or sessions from the training data, as well as an approach that tested for consistency across repeated applications.
Already, preceding physiological changes have been demonstrated to be useful in identifying aggression toward others among youths with autism in a specialized inpatient psychiatry unit. The present work extends this initial research by evaluating the potential for predicting a broader range of aggressive behaviours, including self-harm and emotional outbursts, according to the investigators. [Autism Res 2019;12:1286-1296]
The present study used wearable biosensor data from 70 young participants (mean age 11.9 years, 88.6 percent boys, 90.0 percent White) with autism across four psychiatric inpatient units (mean duration of inpatient hospital stay 37.28 days). Thirty-two participants (45.7 percent) were minimally verbal, and 30 (42.8 percent) had an intellectual disability.
A total of 429 naturalistic observational coding sessions were recorded over 497 hours, wherein 6,665 aggressive behaviours were identified, including self-injury (59.8 percent), emotion dysregulation (31.0 percent), and aggression toward others (9.3 percent).
“Our findings may lay the groundwork for developing just-in-time adaptive intervention mobile health systems that may enable new opportunities for pre-emptive intervention. By focusing on reducing the unpredictability of aggressive behaviour, we anticipate that this ongoing research program may enable inpatient youths with autism to more fully participate in their homes, schools, and communities,” the investigators said. [Ann Behav Med 2018;52:446-462]
They shared that further research will be conducted to explore the clinical implications and potential for personalized interventions based on the study’s findings.