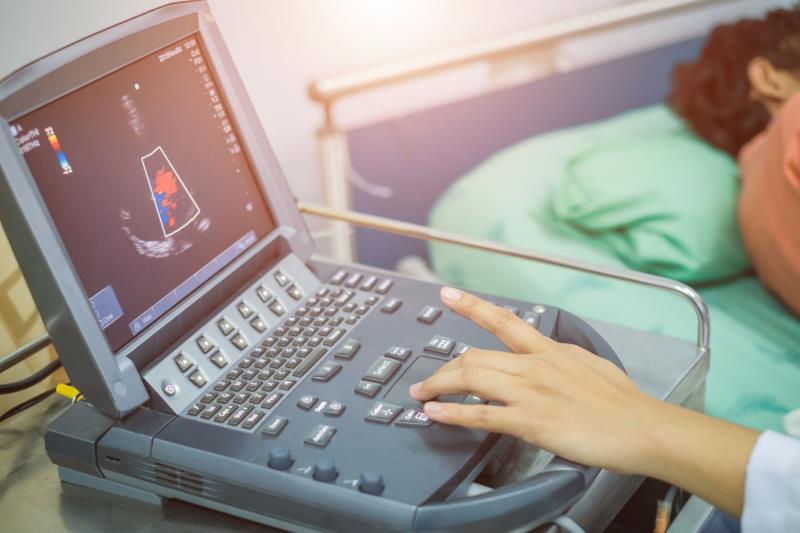
Deep learning interpretation of echocardiographic images demonstrates accurate readings of standard measures of left heart structure and function, which are predictive of cardiovascular (CV) outcomes, reports a recent study.
The researchers developed a deep learning model to interpret echocardiograms and explore the relationship between deep learning-derived echocardiographic measures and incident outcomes. Specifically, the team trained and validated a 3D convolutional neural network model for echocardiographic view classification and quantification of left atrial dimension, left ventricular wall thickness, chamber diameter, and ejection fraction.
A total of 64,028 echocardiograms (n=27,135) derived from a retrospective multi-institutional ambulatory cardiology electronic health record were included in the training sample. Validation was done in a separate longitudinal primary care sample and an external healthcare system dataset. The researchers then used Cox models to examine the association of model-derived left heart measures with incident outcomes.
Deep learning successfully interpreted echocardiographic views (area under the receiver operating curve, >0.97 for parasternal long axis, apical 4-chamber, and apical 2-chamber views vs human expert annotation) and assessed standard left heart measures (R2 range, 0.53 to 0.91 vs study report values). The model showed comparable performance in the two external validation samples.
Left heart measures obtained from the model were able to predict CV outcomes, such as incident heart failure, atrial fibrillation, myocardial infarction, and death. For instance, a 1-SD lower model-left ventricular ejection fraction resulted in a 43-percent higher risk of heart failure (hazard ratio [HR], 1.43, 95 percent confidence interval [CI], 1.23‒1.66) and a 17-percent higher risk of death (HR, 1.17, 95 percent CI, 1.06‒1.30). Results for other model-derived left heart measures were similar.
“Deep learning may enable automated echocardiogram interpretation and disease prediction at scale,” the researchers said.