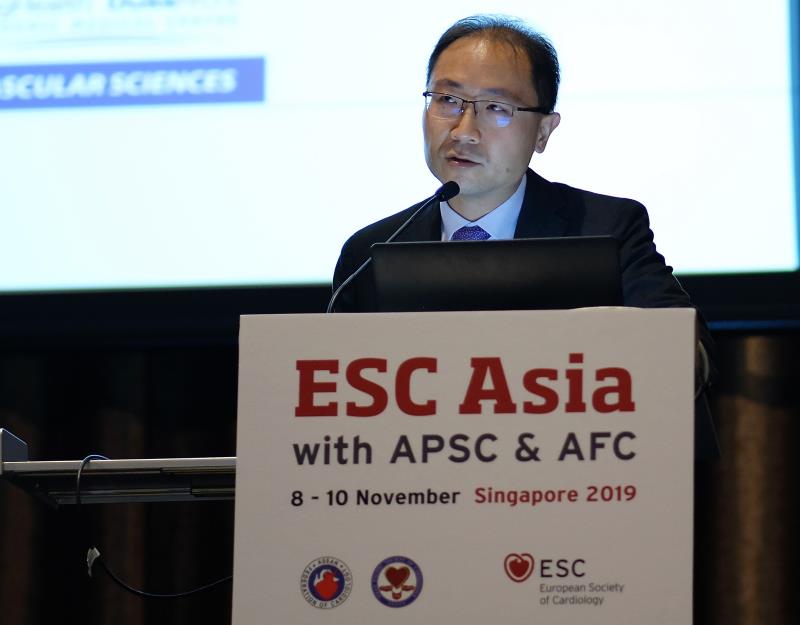
Artificial intelligence (AI) is poised to play a major role in cardiology, according to a recent presentation at the Asia Congress of the European Society of Cardiology (ESC Asia) 2019 in Singapore.
“In practical terms, AI is the ability of a machine, or device, to make autonomous decisions based on data it collects,” says Khung Keong Yeo, adjunct associate professor and senior consultant with the Department of Cardiology at the National Heart Centre Singapore.
“In medicine, this typically involves data being used to predict a likely diagnosis, identify a new disease or select a best choice of treatment,” he added.
According to Yeo, AI can be utilized as a diagnostic tool, particularly for image acquisition, image analysis, and image interpretation or diagnosis. As such, AI can make a diagnosis even without humans.
Recent studies have demonstrated the benefits of implementing AI in cardiology. For instance, Cook and colleagues have shown that AI of instantaneous wave-free ratio pressure-wire pull back data is noninferior to expert human interpretation in determining both the haemodynamic appropriateness for percutaneous coronary intervention (PCI) and the optimal physiological strategy for PCI. [J Am Coll Cardiol 2019;12:1315-1324]
In another study, Howard and his team have found that arterial waveform analysis using neural networks allows rapid and accurate identification of damping, highlighting how machine learning can assist with patient safety and the quality control of procedures. [J Am Coll Cardiol 2019;12:2093-2101]
Yeo, however, cautions about certain pitfalls of implementing AI, which include changing data over time—leading to unstable predictions—false positives and the apparent difficulty in knowing when performance will change, among others.
Moreover, big data solutions are still not yet connected to healthcare, and if social uses and clinical practices are not considered, big data “will fail to cure the health system.”
“We now have even more data,” Yeo says. “Most of the data are not in the clinical, hospital space; they’re outside. Patients are generating the data, and we need to know what to do with it. And I think physicians need to be involved with this.”
The professor further stresses the need to collect large amounts of “good quality data;” to build data science teams or departments, which will include architects, engineers, translators, analysts and overlords; and cross-train physicians in data science.
In addition, he recommends the establishment of good governance on data use and, ultimately, implementation of data science and AI in health systems.
“What we want is augmented intelligence and not just AI,” Yeo says. “We need both.”
Several challenges will have to be dealt with to fully maximize AI, primary of which is the legal framework as well as data quality. Yeo also asks the following questions regarding AI: How quickly can platforms adapt to abrupt changes? How do we train our new doctors? Who owns data? The hospital? The doctor?
“Artificial or augmented intelligence is here, and it will stay. The role in medical care is very probable. Doctors need to learn about data science and AI. Training paradigms will have to adapt,” Yeo says.