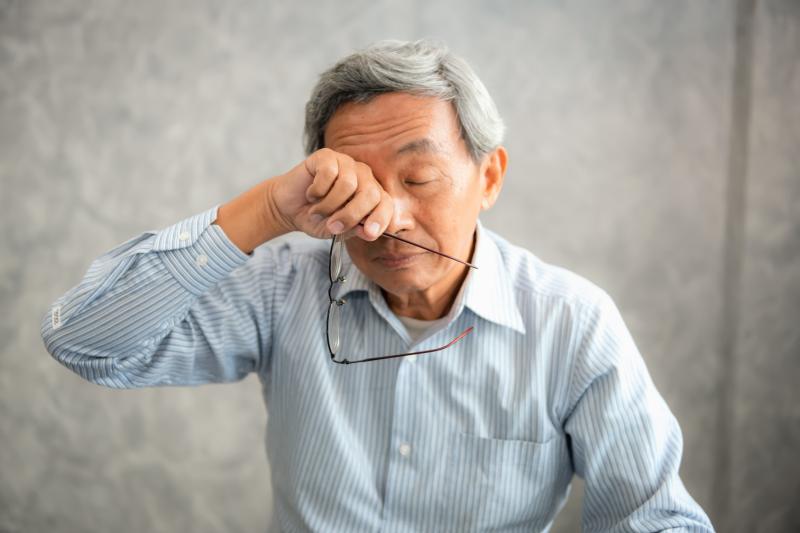
Existing machine learning classifiers for age-related macular degeneration (AMD) demonstrate promising diagnostic accuracy, reports a recent meta-analysis.
Drawing from the online databases of Medline, Embase, ProQuest, and CINAHL, the researchers synthesized evidence from 13 eligible studies encompassing 64,798 participants and 612,429 eye images. Only those that assessed artificial intelligence in the form of machine learning, deep learning, or neural networks for automized AMD diagnosis were included.
Hierarchical summary receiver operating characteristic curve analysis revealed that machine learning classifiers had excellent sensitivity for AMD, with a summary estimate of 0.918. Sensitivity was likewise high at an estimate of 0.888.
According to the researchers, these findings indicated that machine learning could accurately identify 91.8 percent of AMD cases while correctly classifying 88.8 percent of those without AMD as being negative for the disease.
In addition, the positive and negative likelihood ratios were 8.22 and 0.09, respectively, suggesting that using machine learning classifiers was more likely to lead to a positive vs negative diagnosis of AMD.
Moreover, machine learning had a diagnostic odds ratio of 89.74 (95 percent confidence interval, 3.05–2,641.59) for AMD, which meant that AMD patients were 89.74 times more likely to be positively vs negatively screened when using machine learning.
In terms of quality, most studies had a low risk of bias in terms of patient selection (64 percent), flow and timing (100 percent), reference standard (64 percent), and index tests (71 percent).
“To our knowledge, this is the first meta-analysis to analyse the diagnostic accuracy of machine learning classifiers for AMD among all eyes available in datasets to assess their accuracy and reliability to be implemented in clinical settings,” the researchers said.
“The included studies show promising results for the diagnostic accuracy of the ML classifiers for AMD and its implementation in clinical settings,” they added.