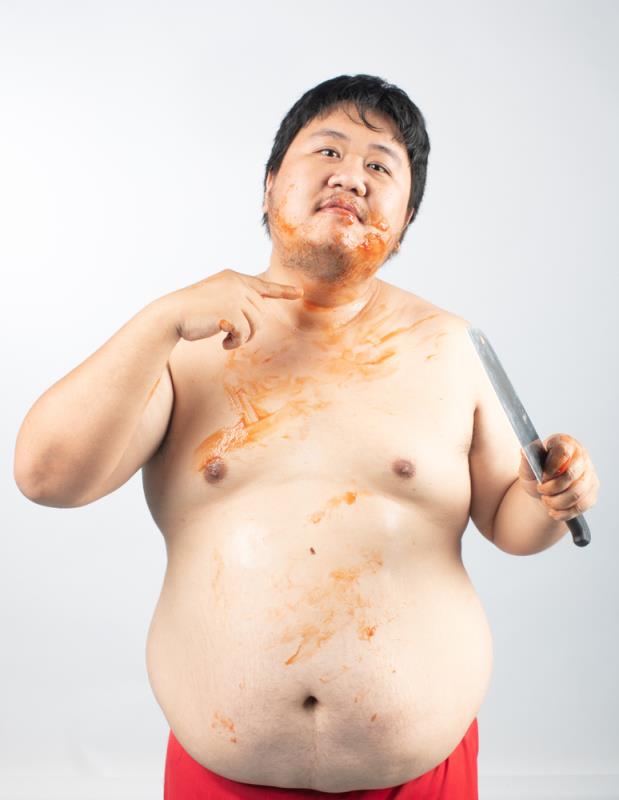
Experts in the UK have recently developed an age-appropriate algorithm for predicting the risk of incident metabolic syndrome, a precursor of cardiometabolic morbidity and mortality, in young adults with psychosis.
“We believe that the age-appropriate algorithm, PsyMetRiC, developed in the study has the potential to become a valuable resource for healthcare professionals working in early intervention service [EIS] by enabling personalized, informed decisions regarding the choice of antipsychotic medications, cardioprotective drugs and lifestyle interventions in preventing the development of cardiometabolic comorbidities and years of life lost for young individuals aged 16–25 years with psychosis,” suggested the authors. [Lancet Psychiatry 2021;8:589-598]
Two PsyMetRiC versions, including the full model (which incorporated factors of age, sex, ethnicity, body mass index, smoking status, prescription of a metabolically active antipsychotic medication, high-density lipoprotein concentration, and triglyceride concentration) and a partial model (which excluded biochemical results) were developed using pooled retrospective data from Birmingham psychosis EIS (n=352) and Cambridgeshire and Peterborough Assessing, Managing and Enhancing Outcomes (CAMEO) EIS (n=299) collected between 1 January 2012 and 4 November 2020. The models were externally validated using data from South London and Maudsley NHS Foundation Trust EIS collected between 1 January 2012 and 3 June 2020. A sensitivity analysis was performed using data from individuals in the Avon Longitudinal Study of Parents and Children (ALSPAC) birth cohort who had or were at risk of developing psychosis (n=505).
The PsyMetRiC model performed well in both internal validation (full model: C 0.80; 95 percent confidence interval [CI], 0.74 to 0.86; partial model: 0.79; 95 percent CI, 0.73 to 0.84) and external validation (full model: 0.75; 95 percent CI, 0.69 to 0.80; partial model: 0.74; 95 percent CI, 0.67 to 0.79).
Calibration plots showed good agreement between observed and expected risk in the full model, but with evidence of slight miscalibration in the partial model.
At a cut-off score of 0.18, the full PsyMetRiC model improved net benefit by 7.95 percent (95 percent CI, 5.37 to 10.82) with a sensitivity of 75 percent and specificity of 74 percent, equivalent to detecting an additional 47 percent of metabolic syndrome cases.