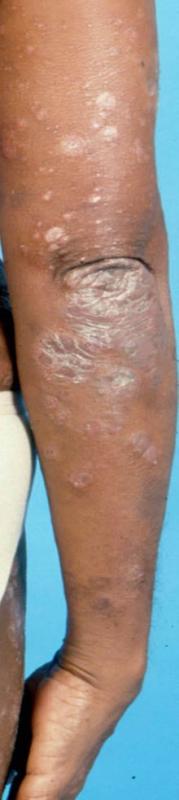
Predictive models that include blood inflammatory proteins such as interleukins (IL) 17A and 17C may help gauge the clinical response of psoriasis patients to tofacitinib and etanercept, a new study has shown.
“From a precision/personalized medicine perspective, this study has further demonstrated the potential of machine-learning to predict treatment responses to well-established psoriasis treatments, and to do so using minimally invasive blood sampling,” said researchers.
From blood samples of 266 patients with moderate-to-severe chronic plaque psoriasis, researchers quantified longitudinal protein profiles of 92 inflammation-associated proteins and 65 cardiovascular disease-associated proteins. A patient was deemed to have responded to the treatment if their Psoriasis Area & Severity Index (PASI) score decreased by >75 percent after 12 weeks of treatment.
Machine-learning algorithms produced several predictive profiles for treatment response. The best model yielded a 78.4-percent accuracy for tofacitinib response, with an area under the receiver operating characteristic (AUROC) curve of 83 percent.
In comparison, the best-performing model for etanercept response had an accuracy of 77.5 percent, with an AUROC of 81 percent.
Both models consisted of inflammatory and cardiovascular disease biomarkers, such as IL-17C, IL-20, IL-12-receptorB, interferon gamma, C–C motif chemokine ligand 20 and C–X–C motif chemokine ligand 10.
Despite promising findings, the present study was conducted on a small cohort of patients and might have issues about generalizability. Further analyses on larger, more representative cohorts are needed.