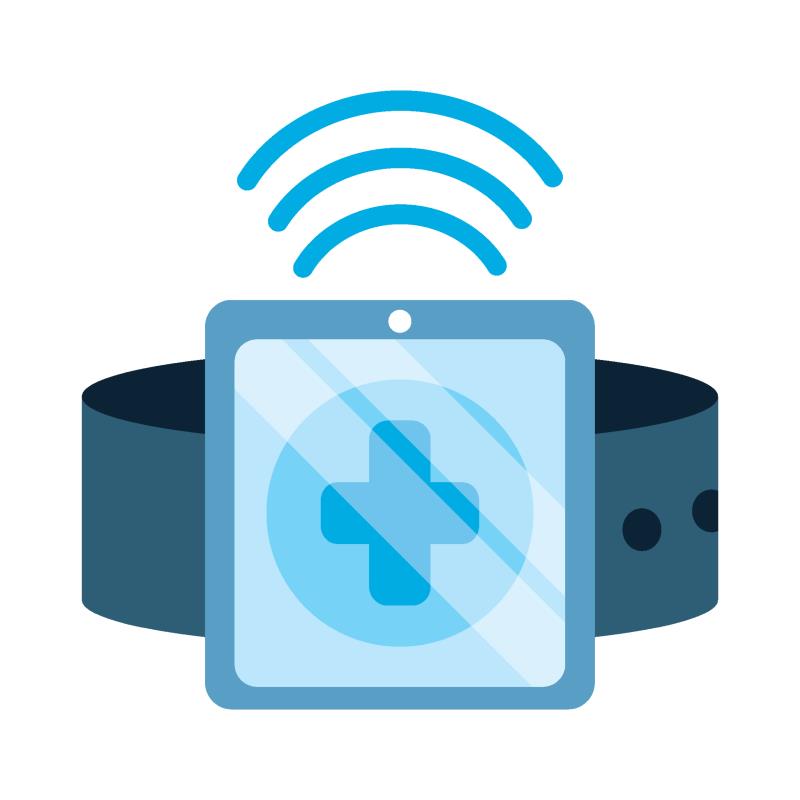
Detecting a broad spectrum of motor and nonmotor seizures is possible using a wrist-worn sensor that integrates custom-developed machine learning (ML) algorithms, according to a team of researchers in Australia.
“Seizures are associated with altered autonomic nervous system activity, manifesting as system changes, including changes in heart rate, blood pressure, respiration, and sweating responses,” said lead researcher Jianbin Tang of IBM Research Australia. [Epilepsy Curr 2004;4:43-46]
“Monitoring of such physiologic signals may allow for tracking of seizure-related autonomic changes and seizure detection,” and ultimately improve outcomes, prevent injury, and potentially reduce the risk of sudden unexpected death in epilepsy (SUDEP), Tang added. [Neurology 2012;78:1868-1876]
ML, particularly deep learning models, has been shown to be useful for automatically detecting, classifying, and predicting epileptic seizure episodes in electroencephalography (EEG) signals. Earlier studies made use of data from electrodes implanted in the brain, but they have increasingly been followed with less intrusive systems measuring scalp EEG data. [NPJ Digital Med 2020;3:59; Lancet Neurol 2013;12:563-571; EBioMedicine 2018;27:103-111]
“The advent of commercially available medical-grade wearable sensors and mobile smart devices, such as smartwatches, provided an opportunity to assess the suitability of other data modalities for seizure detection,” Tang noted. [Seizure 2016;40:88-101]
In the study, Tang and his team asked 94 patients (median age 9.9 years, 57.4 percent female) admitted to the epilepsy monitoring unit of Boston Children's Hospital to wear a sensor around either the left or right wrist or ankle. The device continuously recorded body temperature, electrodermal activity (EDA), accelerometry (ACC), and photoplethysmography to measure blood volume pulse (BVP).
The cohort contributed to 11,066 hours of sensor data, which included 548 epileptic seizures and nine seizure types. These data facilitated ML training and validation for two different algorithms: one was for developing seizure type-specific detection models for nine individual seizure types (algorithm 1) and the other for detecting type-agnostic generalized seizure, with seizure types lumped together (algorithm 2).
Algorithm 1 was able to detect eight of nine seizure types better than chance (area under the receiver operating characteristic curve [AUC-ROC], 0.648–0.976). The same was true for algorithm 2, which detected all nine seizure types better than chance (AUC-ROC, 0.642–0.995). [Epilepsia 2021;doi:10.1111/epi.16967]
When testing different combinations of sensor modalities—such as ACC plus EDA, ACC plus BVP, EDA plus BVP, and ACC, BVP, plus EDA—the fusion of ACC and BVP modalities achieved the best AUC-ROC (0.752) when applied to the entire dataset consisting of all seizure types combined.
Tang, however, stressed that the results should be interpreted in the setting of data acquisition. “Specifically, we included paediatric patients admitted for in-hospital long-term EEG monitoring; activities and behaviours may differ from those at home and manifest differently in wearable device signals.”
Furthermore, he acknowledged that they collected signals from one or two devices, depending on availability, placed on the left or right wrist or ankle. This might have affected the consistency of the signals, since different parts and sides of the body may generate signals that differ in quality and characteristics.
Despite the limitations, Tang believed that wrist-worn and commercially available sensors, running advanced deep learning models and data preprocessing techniques, could be a feasible “out-of-the-box starting alternative” to custom-developed monitoring devices.
“[F]uture work will focus on developing advanced deep learning architectures and data preprocessing schemes individually for each data modality and integrating additional clinical features. We expect that this will allow us to leverage clinical and data modality-related features, thus further improving detection performance and cross-patient generalizability of detection models,” he said.