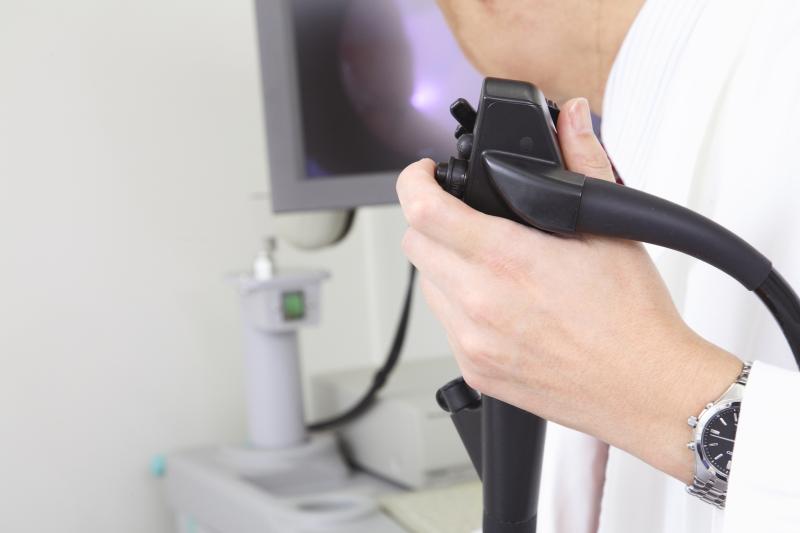
Artificial intelligence (AI) can be harnessed to improve time to diagnosis in capsule endoscopy (CE) workflows, as shown in the use of deep learning models developed by researchers in Singapore.
“Our work serves as reference for future developments in the use of AI in CE workflows—such as in characterizing lesion size, relative location, and capsule small bowel transit times—beyond the demonstrated feasibility in abnormality detection and bowel view quality classification,” said lead researcher Bochao Jiang, Department of Gastroenterology and Hepatology, Changi General Hospital.
Jiang and colleagues demonstrated the use of deep learning models in CE image analysis. Specifically, they piloted a bowel preparation model (BPM) and an abnormality detection model (ADM) to define the frame-level view quality and to identify the presence of abnormal findings, respectively.
In addition, convolutional neural network-based models pretrained on large-scale open-domain data were used to obtain the spatial features of CE images. The researchers used these features in a dense feed-forward neural network classifier. Finally, they combined the open-source Kvasir-Capsule dataset (n=43) and locally collected CE data (n=29).
The performance of the models was compared by averaging five-fold and two-fold cross validation for BPMs and ADMs, respectively. [Singapore Med J 2024;65:133-140]
Based on a pretrained ResNet50 architecture, the best BPM model showcased an area under the receiver operating characteristic of 0.969 and precision-recall curves of 0.843. On the one hand, the best ADM model had top-1 and top-2 accuracies of 84.03 and 94.78, respectively.
The models were capable of processing 200‒250 images per second while demonstrating good discrimination on time-critical abnormalities such as bleeding.
“Although most of this research aimed to provide an augmented decision support capability, there exist several hurdles to address before these algorithms can be formally implemented into daily CE workflows,” Jiang said.
“The core to crossing these hurdles relies on demonstrating their accuracy, sensitivity, and specificity in identifying both common and rare pathologies; addressing class imbalance, conducting temporal, and multi-site validation; and demonstrating transparency in prediction uncertainty,” Jiang added.
Timely detection
Since most CE examinations are carried out on occult small bowel lesions that cause anaemia or suspected small bowel bleeding, a fast and accurate detection of vascular lesions or bleeding is vital, according to the researchers.
Similar studies that developed models to detect bleeding-related abnormalities acknowledged the importance of timely diagnosis, “with each model performance specific to the respective proprietary data used.” [J Med Syst 2011;35:1477-1484; IEEE J Biomed Health Inform 2016;20:624-630; J Exp Theor Artif Intell 2021;33:577-599; IEEE Access 2020;8:132850-132859; IEEE Access 2021;9:33675-33688]
The current study has certain limitations, such as its retrospective, single-centre design and the small number of patients assessed. In addition, the deep convolutional neural network models have been developed using still frames, which do not utilize the rich context provided in the temporal dimension of CE videos. [J Med Biol Eng 2022;42:157-168]
“The value of our work can be further evaluated in a pragmatic manner that is sensitive to existing clinician workflow and resource constraints,” Jiang said.