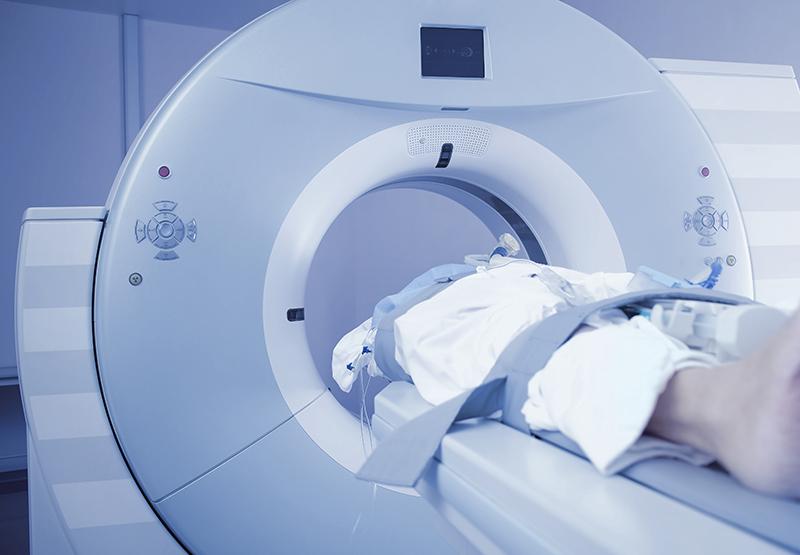
A deep learning-based artificial intelligence (AI) boasts accurate detection of COVID-19 and distinguishes it from community-acquired pneumonia (CAP) and other lung diseases, as shown in a recent study.
Named the COVID-19 detection neural network (COVNet), the 3D deep learning framework extracts both 2D local and 3D global representative visual features from volumetric chest computed tomography (CT) exams.
“The extracted features from all slices are then combined by a max-pooling operation. The final feature map is fed to a fully connected layer and softmax activation function to generate a probability score [for detecting COVID-19],” the investigators said.
“We were able to collect a large number of CT exams from multiple hospitals, which included 1,296 COVID-19 CT exams. More importantly, 1,735 CAP and 1,325 nonpneumonia CT exams were also collected as the control groups in this study in order to ensure the detection robustness considering that certain similar imaging features may be observed in COVID-19 and other types of lung diseases,” they added.
The collected dataset, split into a training set and a testing set, were obtained from 3,322 patients. There was a male predominance across COVID-19, CAP and nonpneumonia groups. Patients with COVID-19 or CAP were much older than those with nonpneumonia diseases for the training (mean age, 53 and 51 vs 41 years; p<0.001) and testing dataset (mean age, 52 and 53 vs 41 years; p<0.001).
COVNet achieved a sensitivity of 90 percent and specificity of 96 percent for detecting COVID-19, with an area under the receiver operating characteristic curve (AUROC) of 0.96. The corresponding values for detecting CAP were 87 percent and 92 percent, with an AUROC of 0.95. [Radiology 2020;doi:10.1148/radiol.2020200905]
“Once the deep learning model is trained, it is very fast to process a new testing exam. The average processing time for each CT exam is 4.51 seconds on a workstation (GPU NVIDIA Quadro M4000 8GB, RAM 16GB, and Intel Xeon Processor E5-1620 v4 @3.5GHz),” they pointed out.
Currently, reverse-transcription polymerase chain reaction (RT-PCR) is considered the reference standard for confirming COVID-19. However, some reports state that the sensitivity of such an approach might not be high enough. [Radiology 2020;doi:10.1148/radiol.2020200642; Radiology 2020;doi:10.1148/radiol.2020200432]
In comparison, CT is a noninvasive imaging approach that has been shown to be capable of detecting certain characteristic manifestations in the lung associated with COVID-19, such as ground-glass opacification, consolidation, bilateral involvement, peripheral and diffuse distribution. [Radiology 2020;295:202-207; Lancet 2020;395:497-506]
Therefore, COVNet could be used as a reliable and rapid approach for screening of COVID-19, according to the investigators. It holds enormous potential for facilitating early diagnosis, isolation and treatment, hence contributing to the control of the pandemic.
The study had several limitations, including the absence of comparison to other viral pneumonias, as well as the lack of transparency and interpretability associated with all deep learning methods.
“As a next step, it would be important to not only predict the presence of COVID-19, but also the severity degree to further help monitor and treat patients,” the investigators said.