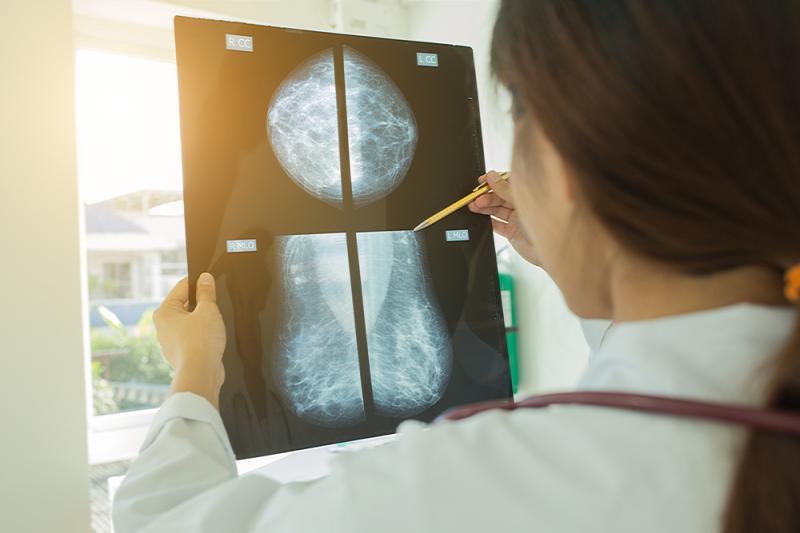
An artificial intelligence (AI) system can predict breast cancer in mammograms better than a radiologist, with results holding across two large datasets that are representative of different screening populations and practices, as shown in a recent study. This highlights the technology’s potential to enhance the efficiency of breast cancer diagnosis.
“Despite the widespread adoption of mammography, interpretation of these images remains challenging. The accuracy achieved by experts in cancer detection varies widely, and the performance of even the best clinicians leaves room for improvement,” the investigators pointed out. [Radiology 2009;253:641-665; JAMA Intern Med 2015;175:1828-1837]
“False positives can lead to patient anxiety, unnecessary follow-up and invasive diagnostic procedures. Cancers that are missed at screening may not be identified until they are more advanced and less amenable to treatment. [This said], AI may be uniquely poised to help with th[e] challenge,” they added. [JAMA Intern Med 2014;174:954-961; NPJ Breast Cancer 2017;3:12]
The AI system used in the study consisted of an ensemble of three deep learning models, each operating on a different level of analysis (individual lesions, individual breasts and the full case). It was trained and tuned using mammograms from about 76,000 women in the UK (where screening is performed every 3 years) and more than 15,000 in the US (where women are screened every 1–2 years). The system's performance in the clinical setting was evaluated in UK and US test sets that comprised 25,856 and 3,097 women, respectively.
Relative to the diagnosis made by first or sole radiologists, the AI system reduced the rates of false-positive and false-negative detection of biopsy-confirmed breast cancers by 1.2 percent and 2.7 percent, respectively, in the UK test set, and by 5.7 percent and 9.4 percent in the US dataset. [Nature 2020;577:89-94]
The system was remarkably able to generalize across populations and screening settings. When trained using only the UK dataset and applied to the US test set, the AI likewise outperformed radiologists, improving both the specificity (3.5 percent; p=0.0212) and sensitivity (8.1 percent; p=0.0006) of breast cancer diagnosis.
Furthermore, in an independent study of six radiologists, the AI system emerged as superior to all human readers. The area under the receiver operating characteristic curve achieved with the AI system was greater by an absolute margin of 11.5 percent compared with that achieved with the average radiologist.
The AI system maintained a noninferior performance when used to provide a second opinion as part of the double-reading process used in the UK, and it reduced the workload of the second reader by 88 percent. This result specifically underscores the potential of AI “to alleviate pressures on services in the context of a worldwide shortage of radiologists,” according to the investigators.
Overall, the data indicate that an AI system is capable of surpassing human experts in breast cancer prediction, they added.
“The optimal use of the AI system within clinical workflows remains to be determined. The specificity advantage exhibited by the system suggests that it could help to reduce recall rates and unnecessary biopsies. The improvement in sensitivity exhibited in the US data shows that the AI system may be capable of detecting cancers earlier than the standard of care. An analysis of the localization performance of the AI system suggests it holds early promise for flagging suspicious regions for review by experts,” they pointed out.
Beyond improving reader performance, the technology could also do away with double reading in most of UK screening cases, while maintaining a similar level of accuracy to the standard protocol, the investigators said. This points to the potential of delivering screening results in a sustainable manner despite workforce shortages.
“Prospective clinical studies will be required to understand the full extent to which this technology can benefit patient care,” they added.