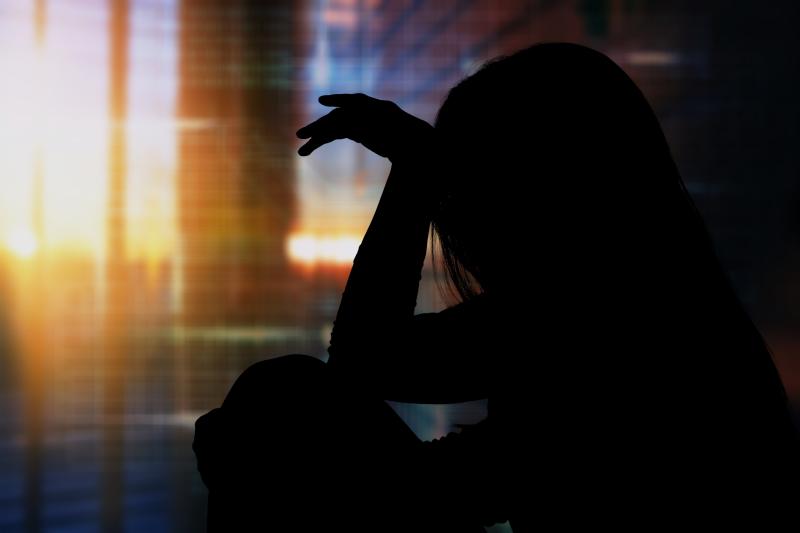
By using digital biomarkers that reflect behavioural and physiological patterns, consumer-grade wearable devices have the potential to screen for people with or at risk of depression, according to a recent Singapore study.
“We found that greater severity of depressive symptoms was robustly associated with greater variation of night-time heart rate between 4 AM and 6 AM; it was also associated with lower regularity of weekday circadian activity rhythms based on steps and measured with nonparametric measures of interdaily stability (IS) and autocorrelation,” the researchers said.
Nevertheless, predictive ability of the digital biomarkers remains limited, particularly in the whole sample, underscoring the need for further research.
The cross-sectional analysis included 267 health working adults (mean age 33±8.6 years, 63.7 percent women) who were asked to wear Fitbit Charge 2 devices for 14 consecutive days. Scores in the 9-item Patient Health Questionnaire (PHQ-9) showed a gradual and linear decrease in depressive symptoms over time. [JMIR Mhealth Uhealth 2021;9:e24872]
Based on a PHQ score ≥10 either at baseline or at the follow-up assessment 2 weeks later, 38 participants (14.2 percent) were deemed to be depressed.
Correlation analysis initially identified 36 digital biomarkers that were correlated with average PHQ-9 scores, of which only 17 remained after false discovery rate correction. Eleven biomarkers remained robust after linear regression analysis, though only three eventually emerged as putative predictors of the severity of depressive symptoms independent of all confounders.
These predictive biomarkers included the coefficient of variation (CV) of heart rate between 4 AM and 6 AM, weekday steps-based IS, and autocorrelation based on 15-minute intervals.
In particular, both steps-based IS (β per 10-percent change, –0.30, 95 percent confidence interval [CI], –0.51 to –0.08; p=0.01) and autocorrelation (β per 10-percent change, –0.38, 95 percent CI, –0.75 to –0.01; p=0.04) were significantly and inversely correlated with PHQ-9 scores, suggesting that “participants with lower stability of circadian activity rhythms had more severe symptoms,” the researchers explained.
In contrast, CV of heart rate between 4 AM and 6 AM was positively associated with PHQ-9 (β per 10-percent change, 0.90, 95 percent CI, 0.04–1.78; p=0.04), indicating that greater variations in heart rate could signal worse depressive symptoms.
“Despite several reliable associations, our evidence showed limited ability of digital biomarkers to detect depression in the whole sample of working adults,” the researchers pointed out.
“However, in balanced and contrasted subsamples comprised of provisionally depressed participants and healthy participants with no risk of depression, the model achieved an accuracy of 80 percent, a sensitivity of 82 percent, and a specificity of 78 percent in detecting subjects at high risk of depression,” they added.
Such findings highlight the need for longitudinal validation and the development of semipersonalized models of depression prediction, which could take into consideration different baseline characteristics to predict depression risk more accurately. Moreover, “wearable sensor data can be enriched with smartphone data, which will enable more comprehensive digital phenotyping for depression detection,” the researchers said.