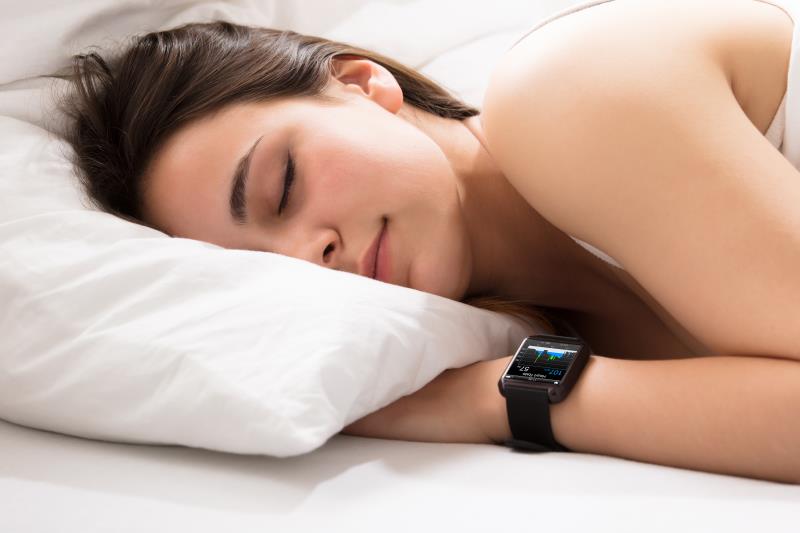
While wearable fitness trackers have the potential to provide accurate sleep and heart rate (HR) estimates in shift workers under naturalistic conditions, crucial limitations of the technology become apparent when compared against the gold standard of polysomnography (PSG), even after careful data treatment, according to a recent study
“The generally wide limits of agreement hamper the precision of quantifying individual sleep episodes. The value of this consumer-grade multisensory wearable in terms of tackling clinical and research questions could be enhanced with open-source algorithms, raw data access, and the ability to blind participants to their own sleep data,” the researchers said.
The study included 59 shift workers (mean age 33.5±8.1 years, 56 percent women) who were asked to collect two nights’ worth of ambulatory PSG recordings. Participants were free to decide on their sleep time and duration. Concurrent with PSG nights, participants were also asked to wear the Fitbit Charge 2 device. The agreement between two sleep assessment methods was assessed using Bland-Altman plots and on an epoch-by-epoch basis.
Compared with PSG, the Fitbit device overestimated rapid eye movement latency (REML) by 29.4 minutes, and wake after sleep onset (WASO) by 37.1 minutes (p<0.001 for both). The Fitbit also slightly underestimated stage 3 non-REM sleep by around 11 minutes (p=0.08). [J Med Internet Res 2021;23:e26476]
In contrast, no such bias vs PSG were found for sleep onset and offset, duration of REM sleep, combined duration of stage 1 and 2 non-REM sleep, and duration of stage 3 non-REM sleep; however, limits of agreement (LoA) were non-negligible.
Moreover, the marginal distributions of the Fitbit-measured duration of deep, light, and REM sleep showed high variance even after excluding outliers. “This observation may indicate that the estimation of stages of sleep is challenging for Fitbit’s algorithm and a source of variability, although being unbiased,” the researchers explained.
“The unbiased sleep variables allow average estimations of important sleep quality characteristics in ecological conditions,” they added, pointing out that the utility of such devices for the collection of reliable sleep data could be hampered by the large biases observed in REML and WASO, as well as the generally wide LoAs for most variables.
In terms of HR data, Fitbit returned a slight underestimation (0.9 bpm) of the 10 percent-trimmed heart rate average (HR10), and a strong and significant underestimation of the variance of HR10 (20.3 bpm; p<0.001).
Overall, the findings of the present study “highlight the considerable challenges still present when relying on consumer-grade technology to address clinical and research questions,” the researchers said.
“Furthermore, future validation studies should also be conducted in populations with sleep disorders, such as narcolepsy, who often present with … sleep episodes that appear particularly difficult to detect. Such studies can help identify the factors that determine the accuracy of Fitbit’s sleep and HR measures,” they added.