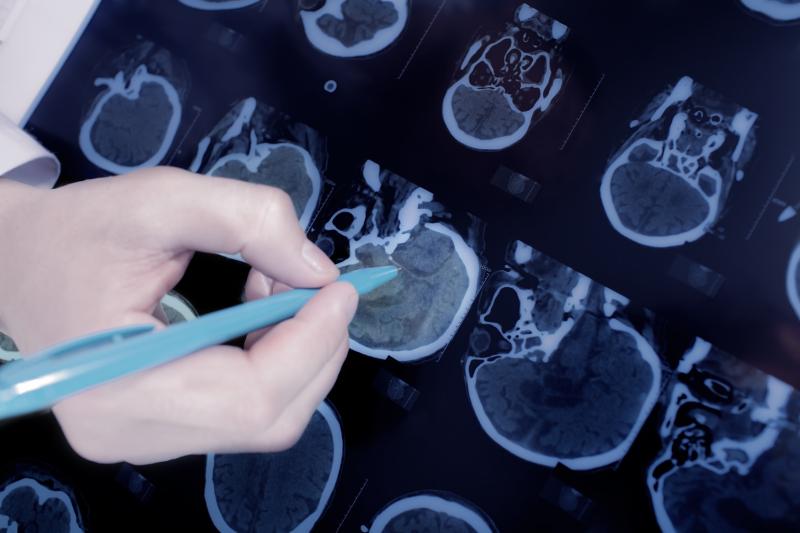
A graph convolutional and recurrent neural network (graph-CNN-RNN) model can identify patients who are likely to develop Alzheimer’s disease (AD) at around 80 percent accuracy, according to a recent Singapore study.
“This study developed the graph-CNN-RNN model and defined a scalar measure, AD probabilistic risk, that well characterizes whole-brain morphology,” the researchers said. “The graph-CNN-RNN was a longitudinal model that incorporated longitudinal cortical and subcortical morphology for the diagnosis and prediction of AD.”
The present study used data from the Alzheimer’s Disease Neuroimaging Initiative (ANDI; n=2,491) and the Open Access Series of Imaging Studies-3 (OASIS-3; n=930), two of the largest AD datasets, both of which used several timepoints. Half of the ANDI (n=1,559) dataset was used to train the graph-CNN-RNN model, which was in turn tested on the other half of ANDI and the entire OASIS-3.
The resulting graph-CNN-RNN longitudinal model was able to accurately classify stable controls and AD patients of the ANDI dataset, with accuracy rates ranging from 85.4 percent to 92.3 percent over 6 years from baseline. [Neuroimage Clin 2022;34:102993]
Directly applying the model to OASIS-3 yielded an accuracy range of 82.6 percent to 95.2 percent over 2 years from the baseline, suggesting that the graph-CNN-RNN model was robust and reliable in classifying stable controls and AD patients.
The researchers then developed a novel scalar measure, called AD probabilistic risk, which was defined as the ratio of the probability of being diagnosed with AD to the sum of the probabilities of having AD and of being cognitively normal (CN).
Models showed that in both data sets the probabilistic risk was highest in AD patients and lowest in controls. Moreover, longitudinal analysis found that the graph-CNN-RNN model could predict AD years in advance.
For example, the accuracy for AD conversion was 79.7 percent even when the model used magnetic resonance imaging scans from 4 years before AD onset. The prediction accuracy was fairly consistent throughout the 4-year period before onset, with values ranging from 78.3 percent to 81.5 percent.
Prediction accuracy was relatively higher when the model was tested on larger sample sizes. Meanwhile, the 95 percent confidence intervals tended to be wider in smaller sample sizes, suggesting larger uncertainties.
"This study offered a promising deep learning tool for individualized diagnosis and prognosis over the course of AD," the researchers said. “Such a deep learning tool and the AD probabilistic risk have great potential in clinical practice.”
“The performance and robustness of the proposed model should be further tested on longitudinal datasets with larger samples,” they added. “Moreover, as multimodal brain image data become available, our model can be extended to other image modalities and create a functional, metabolic, and pathological signature for the diagnosis and prognosis of AD.”