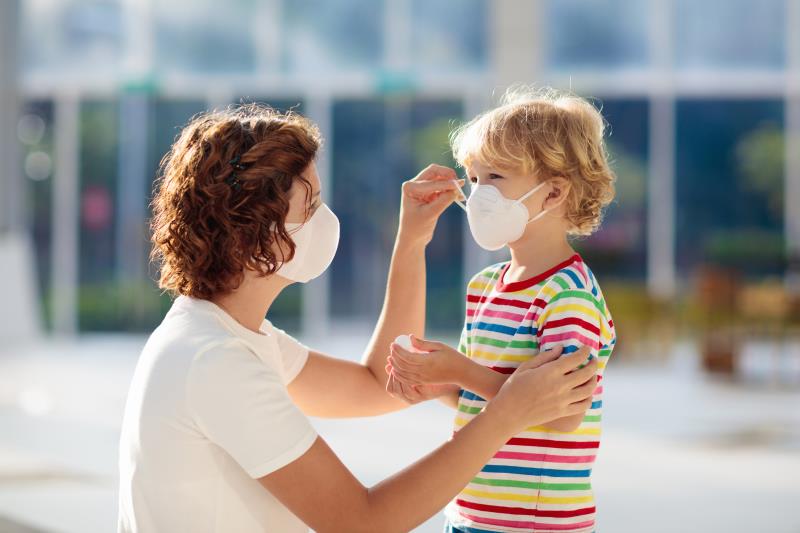
Smartwatches, with the support of machine learning algorithms, can perceive face-touching (FT) behaviours and may help contain outbreaks of respiratory infections such as the coronavirus disease 2019 (COVID-19), according to a new study.
“We utilized state-of-the-art machine learning algorithms: logistic regression, support vector machine, decision tree, and random forest for FT recognition,” the researchers said. “Results demonstrate that machine learning models were accurate at recognizing facial touches. However, models performed less optimally when recognizing individual physical activities.”
Ten participants (aged 20–83, five women) were enrolled and asked to perform 10 physical activities classified as either FT or non-FT. All were given a wrist-worn fitness tracking device to collect raw accelerometer data, which were then fed into the four machine learning methods to discern FT from non-FT movements.
All four machine learning algorithms were able to accurately differentiate FT vs non-FT movements. Logistic regression showed the best performance with a mean accuracy value of 0.93±0.08 and a mean F1 score of 0.90±0.11. In comparison, the Decision Tree algorithm had the poorest performance with corresponding estimates of 0.88±0.09 and 0.84±0.13. [Sensors 2021;21:6528]
Receiver operating characteristic curve analysis confirmed that logistic regression processing of raw device data could reliably differentiate FT from non-FT behaviours.
The researchers then assessed whether device measurements with machine learning processing could detect individual activities, such as writing, eating and drinking, adjusting eyeglasses, and using a computer, among others.
They also found that while machine learning could accurately discern FT behaviours, it had poor individual activity recognition. Random Forest processing, for instance, had the best performance with only 0.70±0.14 mean accuracy and 0.67±0.15 mean F1 score. Decision Tree performed the worst, with corresponding mean estimates of 0.59±0.11 and 0.56±0.12.
Such an effect could be explained through confusion analysis, the researchers said. They generated a Confusion Matrix, which found that the machine learning algorithms were more likely to confuse intracategory movements with each other than intercategory actions. For instance, the models might confuse repeated face touching behaviours with non-FT actions such as performing computer tasks or moving items.
“Summing these activities into categories such as the FT and NFT can help in enhancing the recognition performance metrics,” the researchers said. “Collapsing FT activities into one category in such a way can potentially reduce the risk of transmitting an infectious agent through direct or indirect touch.”
In turn, these findings prove “highly significant during respiratory infection outbreaks as it has a great potential to inhibit individuals from touching their faces which is a transmission vector for respiratory illnesses,” they said.
“It also allows researchers and practitioners to utilize the publics’ personal devices to track and monitor their behaviour in their natural environment and intervene when needed,” the researchers added.