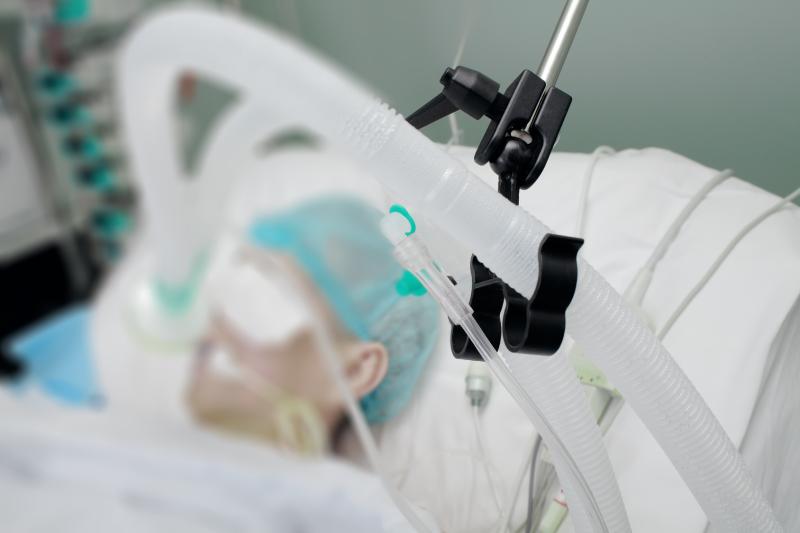
A novel deep learning model has been shown to accurately predict the need for mechanical ventilation among patients with the novel coronavirus disease (COVID-19), reports a study. Prognostic stratification as provided by this model will remain necessary until effective preventive or treatment measures become widely available for these patients.
“To that end, our study demonstrates a proof-of-principle that chest X-ray images acquired early during hospitalization can accurately predict the need for mechanical ventilation in patients with COVID-19,” the researchers said. “Such a tool can be valuable in effectively triaging patients with COVID-19 at the time of initial healthcare contact.”
In this study, the researchers used the well-established DenseNet121 deep learning architecture on 663 X-ray images obtained from 528 hospitalized COVID-19 patients. Two pulmonary and critical care experts blindly and independently assessed these images for validation.
The deep learning model predicted the need for mechanical ventilation with high accuracy (90.06 percent), sensitivity (86.34 percent), and specificity (84.38 percent). Such prediction was carried out about 3 days on average ahead of the actual intubation event. Most notably, the deep learning model outperformed the two experts who evaluated the same X-ray images and provided incremental accuracy of 7.24 percent to 13.25 percent. [BMJ Innov 2021;doi:10.1136/bmjinnov-2020-000593]
“It needs to be mentioned, however, that we do not anticipate that clinicians will use our deep learning model to make clinical decisions,” the researchers said. “[R]ather, the deep learning model is intended to be used as an aid for patient triaging and informed resource allocation by hospitals.”
Prior to this model, the CheXNeXT system has been utilized to predict 14 pathologies based on chest X-rays, but not in the context of COVID-19 infection. Several studies have tried diagnosing COVID-19 based on chest radiographs, but deep learning attempts to prognosticate the disease course have been scarce. [Cognit Comput 2021:1-11; Biomed Eng Online 2020;19:88; Sci Rep 2020;10:19549; Lancet Digit Health 2020;2:e506-515; Biocybern Biomed Eng 2020;40:1391-1405]
Cohen and colleagues developed an algorithm to predict patients at high risk of death, while Zhu and team developed a deep learning method to stage disease severity. Recently, Li and colleagues developed a deep learning Siamese network to predict the Radiographic Assessment of Lung Edema scores used to quantify severity of acute respiratory disease syndrome in COVID-19 patients. [Cureus 2020;12:e9448; PLoS One 2020;15:e0236621; medRxiv 2020;doi:10.1101/2020.05.20.20108159]
“These landmark studies have proffered definitive directions for the potential use of chest X-rays in clinical care of critical patients. However, a direct application of these systems to predict actionable outcomes like the need for mechanical ventilation is currently lacking,” the researchers said.
“In totality, these previous studies provide a possible biological explanation as to why chest radiographs can predict the need for mechanical ventilation in immediate future,” they added.
The current study was limited by retrospective design, potential confounding and bias, data obtained from a single centre only, use of chest radiographs only, use of the Harrel’s C statistic as a measure of the predictive accuracy of the Cox regression models, and the evaluation of images by experts for the purpose of research and not as part of routine patient care.