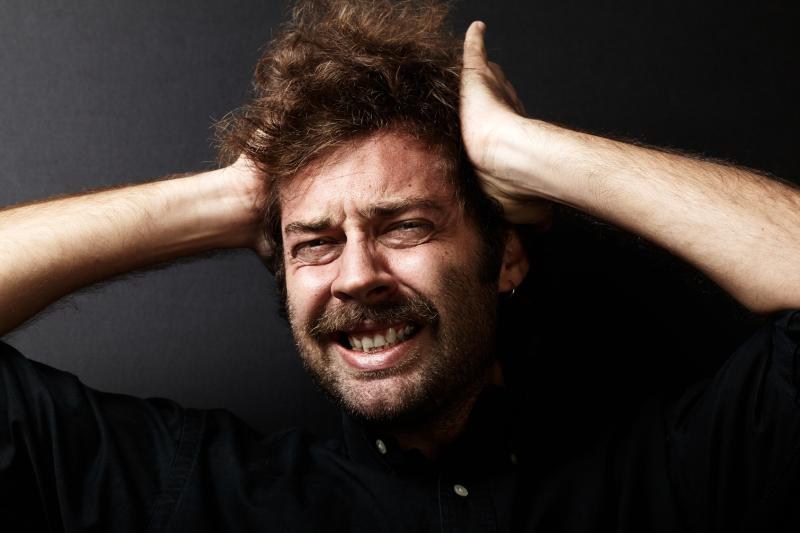
Machine learning models, using data from wearable devices, questionnaires, and the environment, can help predict panic attacks (PA) in the next 7 days, according to a recent study.
“It is possible to predict PAs 7 days before the fact by using a combination of all data from questionnaires, physiological data, and environmental data,” with models reaching an accuracy of over 80 percent, the researchers said. “The prediction performance of the all-feature model is better than the physiological-environment model or questionnaire model alone.”
Fifty-nine participants (mean age 46.2 years, 61 percent women) with panic disorder were enrolled in the study and were given app-paired smartwatches for the assessment of heart rate (HR), sleep, and activity level. Anxiety and depression were evaluated through the Beck Depression and Anxiety Inventories, the State-Trait Anxiety Inventory, and Panic Disorder Severity Scale Self-Report (PDSS-SR). Air Quality Index data were also included.
Six machine learning methods were used to process these data for the prediction of 7-day PA: random forests, decision trees, linear discriminant analysis, adaptive boosting, extreme gradient boosting, and regularized greedy forests.
The training set for the models included 3,249 data points, of which 64.91 percent (n=2,109) indicated no PA according to the PDSS-SR. Meanwhile, 25.61 percent (n=832), 7.11 percent (n=231), 1.79 percent (n=58), and 1.6 percent (n=52) showed mild, moderate, severe, and extreme PA episodes, respectively. [JMIR Med Inform 2022;10:e33063]
Using the training set, the random forest model emerged as the best PA predictor, with an accuracy of 0.975 and an F1 score of 0.968. It was followed closely by the decision tree model (0.949 and 0.936, respectively) and more distantly by the linear discriminant analysis model (0.746 and 0.647, respectively).
The test set included 947 data points, most of which also did not show PA (65.8 percent; n=641). The random forest model was again the best predictor of PA, with accuracy and F1 values of 0.813 and 0.677. Regularized greedy forest (0.800 and 0.660, respectively) and adaptive boosting (0.746 and 0.576, respectively) models also performed well in the test set.
Using different combinations of data sets, the researchers also showed that prediction performance is improved by including all features rather than by focusing on physiological and environmental factors alone, or on questionnaire answers alone.
“To better predict PAs, it is possible to use multifactorial items from clinical questionnaires and physiological and environmental data,” the researchers said. “Among these, clinical questionnaires are more crucial than their physiological-environmental counterparts.”
“It is also beneficial to collect information from baseline anxiety and depression, trait anxiety, the number of comorbid psychiatric diagnoses, the average and resting HR, and deep sleep duration as a reference to predict recurrent PAs for patients with PD,” they added.
Future studies should include larger and more heterogeneous samples, as well as try to achieve PA prediction hours before occurrence, according to the researchers.