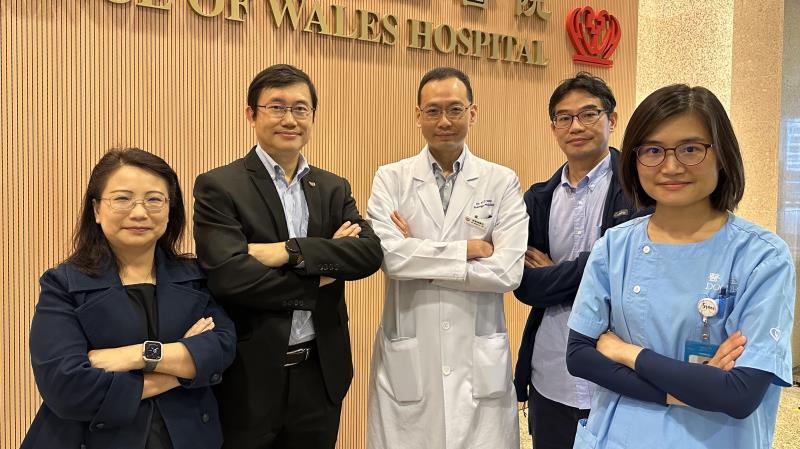
Researchers from the Chinese University of Hong Kong (CUHK) have developed an automatic retinal image analysis (ARIA) technology that utilizes machine learning to improve cardiovascular disease (CVD) risk estimates in people living with HIV (PLWH) who have cardiovascular (CV) risk factors.
Compared with the general population, PLWH have a two-fold increased risk of CVD. [Circulation 2018;138:1100-1112] Regular CVD risk assessment for PLWH is recommended by clinical practice guidelines. [Circulation 2019;140:e98-e124; EACS Guidelines 11.1, https://eacs.sanfordguide.com] “However, traditional CVD risk assessment tools are not tailored for PLWH. An HIV-specific CVD prediction model is needed,” stated Dr Grace Lui of the Department of Medicine and Therapeutics, CUHK.
Retinal characteristics have been identified as useful biomarkers for chronic diseases, such as CVD. [Curr Opin Ophthalmol 2021;32:439-444; Nat Biomed Eng 2018;2:158-164] “[For example,] coronary artery disease [CAD] is associated with arteriolar and venular calibre, curvature tortuosity and branching complexity,” explained Professor Benny Zee of the Jockey Club School of Public Health and Primary Care, CUHK. [Atherosclerosis 2018;268:215-224; Sci Rep 2016;6:19053]
In this study, the researchers evaluated the performance of CVD prediction models based on ARIA alone, traditional CV risk factors alone, and ARIA plus traditional CV risk factors in at-risk PLWH. The ARIA algorithm detected retinal characteristics, including arteriole and venule width, in retinal images captured by digital fundus photography. The participants’ CVD risk was also calculated using established CV risk prediction functions, such as the Framingham risk score. The performance of the three models was compared by the area under the receiver operating characteristic curve (AUC). [PLoS One 2023;18:e0281701]
The study included 115 PLWH (mean age, 53.7 years; male, 88.7 percent; median duration since HIV diagnosis, 12 years) managed at the Prince of Wales Hospital, Hong Kong, between February 2019 and February 2021. At baseline, the participants had either chest pain (17.4 percent) or ≥1 CV risk factor (diabetes, 40.0 percent; hypertension, 45.2 percent; dyslipidaemia, 45.2 percent; obesity, 54.8 percent). CT coronary angiography showed coronary atherosclerosis and obstructive CAD in 61.7 percent and 20.0 percent of participants, respectively.
For coronary atherosclerosis detection, the ARIA model outperformed the model based on traditional CV risk factors alone (ARIA model: AUC, 0.979; 95 percent confidence interval [CI], 0.960–0.998) (traditional model: AUC, 0.746; 95 percent CI, 0.652–0.841). The ARIA model demonstrated sensitivity of 91.5 percent and specificity of 88.6 percent, while the traditional model showed sensitivity of 81.7 percent and specificity of 54.5 percent. Performance was further improved when ARIA was combined with traditional risk factors (AUC, 0.987; 95 percent CI, 0.973–1.00), with sensitivity and specificity of 93.0 percent and 93.2 percent, respectively.
Detection of obstructive CAD was similar between the combination model (AUC, 0.991; 95 percent CI, 0.978–1.000) and ARIA model (AUC, 0.986; 95 percent CI, 0.970–1.000). Both ARIA-based models performed better than traditional risk factors alone (AUC, 0.777; 95 percent CI, 0.672–0.883).
“Our study has demonstrated that machine learning–based retinal image analysis [ie, ARIA] may enhance risk assessment of coronary atherosclerosis and obstructive CAD in high-risk PLWH,” the researchers concluded.