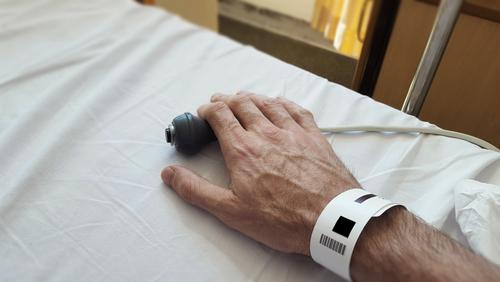
Wristband actigraphy devices, through the help of deep-learning prediction models, can predict survival outcomes in end-stage cancer patients on palliative care, according to a recent study.
“Our survival prediction model provided satisfactory prognostic accuracy of patients’ binary survival outcomes, death or discharged in stable condition, by using activity data of the initial 24 or 48 hours on their hospital admission,” the researchers said. “The prognostic accuracy of the model was time-dependent, with models using activity data of 48 hours yielding better results than those of 24 hours.”
Sixty patients (mean age 72.9 years, 62 percent men) admitted to hospice care were enrolled into the study and given a wrist-worn actigraphy device. The device collected time series data with three features (physical activity, spin, and angle). A long short-term memory (LSTM)-based deep learning model was used to predict whether a patient would die or would be discharged in stable condition.
Twenty-eight patients died during follow-up, yielding a mortality rate of 47 percent; 32 (53 percent) were discharged from hospice care in stable condition. Karnofsky Performance Status (KPS) and Palliative Prognostic Index (PPI) data were available for 59 and 20 participants, respectively, of whom 28 and 8 scored <50 percent and >6.0 on the corresponding indices at baseline. [Front Public Health 2021;9:730150]
Using activity data collected by the wrist device during the initial 48 hours after admission, the preliminary LSTM model showed a predictive accuracy of 0.8667 for survival in the training dataset and 0.7143 in the testing dataset.
The researchers constructed a confusion matrix in order to visualize the differences between the model’s predictions and ground truth. Their matrices showed that without normalization, the LSTM model misclassified 38 percent of discharges as deaths. On the other hand, errors were less likely to occur with deaths, such that only 17 percent of mortalities were misclassified as discharges. Normalization led to better model performance.
The resulting sensitivity and specificity estimates for the LSTM model in the testing dataset were 0.8333 and 0.625, respectively. The obtained area under the curve (AUC) was 0.7292.
“These satisfactory results indicated the feasibility of LSTM in classifying time series data collected by wearable actigraphy devices without any physiological information,” the researchers said.
Increasing the LSTM units from 64 to 256 led to improvements in the model, such that the final sensitivity and specificity values were 1.90 and 0.6667, respectively, with an AUC of 0.8333.
In a subsequent exploratory analysis, the researchers examined the impact of the length of data collection on the model performance. They found that when they shortened the data collection period to 24 hours but applied the same LSTM parameters, the resulting model had poorer prognostic accuracy.
“While the better performance of the model may be attributed to the increasing length of data, the inclusion of at least a cycle of circadian rhythms might also serve as a constructive factor,” the researchers said. “Future studies examining the impact of circadian rhythm on activity data of end-stage cancer patients are thus warranted.”
The wearable actigraphy device used in this study is lightweight and low-cost. Based on the results, it provides convenient activity data for survival prediction in end-stage cancer patients.